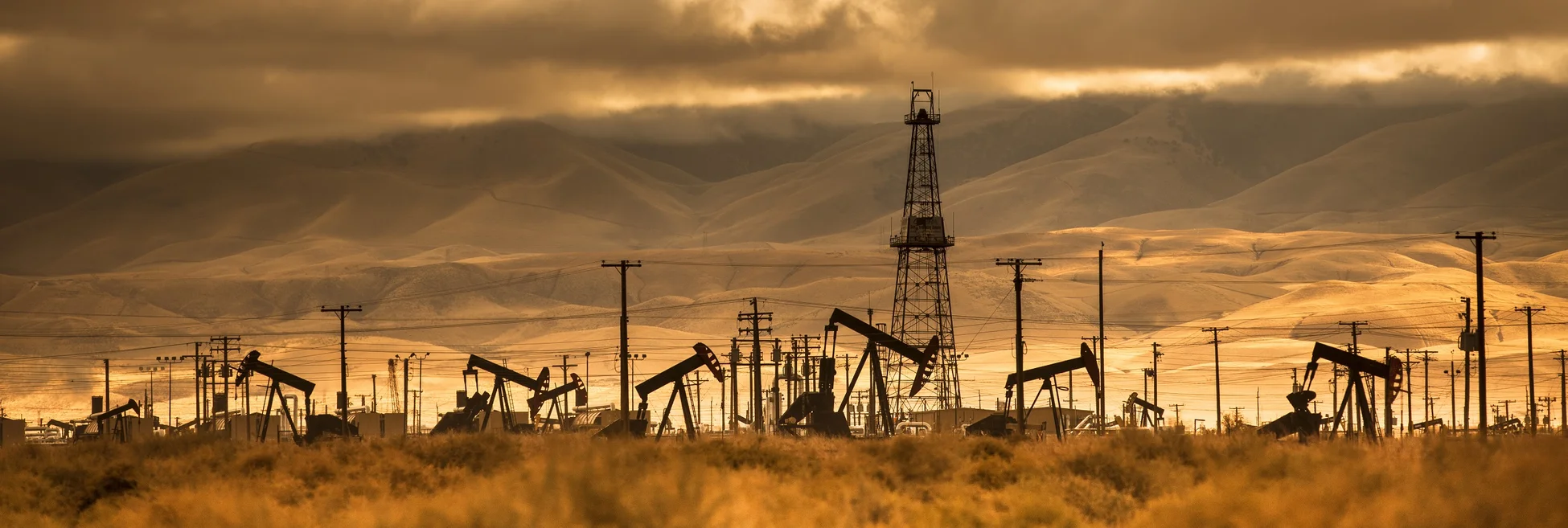
The New Oil Rush: An Examination of the Alternative Data Market
Banks are looking to cash in on the alternative data boom, but an in-depth investigation of the alternative data market shows that they may be in for an uphill battle to claim territory.
From Texas to Pennsylvania, and Calgary on down to the Gulf of Mexico, oil rushes have played a key role in the shaping of North America, bringing with them both vast wealth and colossal despair. The latest oil boom is unfolding in North Dakota. From 1920 to 2009, the state’s population had rested steadily at around 640,000 residents. But as the financial crisis started to unfold and a barrel of oil shot up to $100 per barrel, a relative flood of people entered the state looking to tap into its vast petroleum resources coming from the Parshall Oil Field, which was discovered in 2006. The result was the population increasing by 18 percent to just over 755,000 from 2008 to 2017, after decades of essentially net-zero growth.
In today’s financial markets, data is often referred to as the oil that runs the machine. If that’s indeed the case, then the vast alternative data market represents the Parshall Oil Field at a time when regulation and the challenge of finding new forms of alpha generation are leading to the $100-a-barrel oil boom.
According to the website alternativedata.org, there are 375 alt data providers focused on providing information to institutional investors; in 2013 there were fewer than 250. According to IBM, 90 percent of all alternative data in circulation today was created in the last two years. And capital-markets consultancy Opimas estimates that in 2018, the alternative data market—including data sources, IT infrastructure, system development, and human capital—will exceed $5 billion, and will climb to almost $8 billion by 2020.
This bull market can be attributed to three primary causes: the explosion of available data; the ability to store that data, cheaply; and the growing sophistication of analytics tools that can deliver insights faster at capabilities beyond human cognition. But there’s more to it than data and tech advancements—the revised Markets in Financial Instruments Directive’s (Mifid II) research unbundling component has proven disruptive to the old boys network ways of brokers selling information to the buy side; buy-side firms are relying on the vendor, fund administrator and broker communities to help with their data collection and management needs post-2008; and the rise of passive investing have cut into hedge fund profits, so they need to find new sources of alpha generation.
As a result, the sell side is trying to figure out how to get in on this boom. Citi is creating a consulting team that will work with clients on bespoke alternative data projects; Goldman Sachs is building a team to sell its own internally-created/captured data back to clients; Morgan Stanley is creating a consulting team and application programming interfaces (APIs) that will allow users to feed into curated datasets. It will not be an easy task.
Waters spoke with over two-dozen individuals at a range of institutions—including banks, hedge funds, traditional asset managers, vendors, exchanges and consultants—to better understand the alternative data market. The short-and-skinny of it is that the banks are worried about being left nibbling at the scraps of a burgeoning market. But, in reality, they’re already well behind the vendor community. Data giants like FactSet and Bloomberg have already built out their services. At the same time, the fintech community is proving to be its usual disruptive, nimble self.
Reinventing the Wheel
From Bank One’s ill-fated ownership of Telerate to the bank community creating trade reporting utilities such as BOAT, the sell side has had a shaky history of owning data providers, as they seem to lack the long-term commitment necessary to see these endeavors though.
Several sources laid out this timeframe for bank involvement in the alternative data space, which generally follows a pattern where banks try to figure out a way to sell their own internal data; buy a third-party provider rather than continue to build the team internally, and then spin out that unit after disillusionment sets in. That, or they try and become an alternative data hub, before realizing that there are already a multitude of specialists in the space who do it better, then buy them, et cetera.
“Why become a data vendor and compete with the likes of FactSet, Bloomberg, Refinitiv? It doesn’t make sense,” says Bruce Fador, head of Fador Global Consulting Group. “The buy side is not going to embrace that model. The sell side isn’t exactly unbiased—they can’t become Switzerland and market it as effectively as a vendor can. You’re in the market of selling ideas [through research] and these aren’t ideas.”
A data head at a tier-one US bank echoes Fador’s sentiments.
“My personal view on the alternative data space is that the jury is still out,” says the source. “First of all, a lot of the data is just not going to have enough investment value and no one is going to pay for something that doesn’t improve their signal. Then you’re going to have data that does improve the signal, but the question is, how much are they going to be willing to pay for it? And are the firms that provide that data going to take broad or narrow distribution approaches with it, because obviously the more you distribute it, the less value it has.”
We’ll never replace the need for our buy-side customers to invest in these capabilities on their own, but our goal is to make it easier for them.
Bill Dague, head of alternative data, Nasdaq.
The data manager is also skeptical as to how much many of these alt data signals are improvements over more traditional signals—is the cost worth the noise?
“Asset managers are interested in this data, but there are hundreds of these providers out there, bringing in all of their data, signing a non-disclosure agreement, getting the data in, parsing it, making it usable so you can run your backtest on it, running the backtest… it all takes work and time, and most of our clients don’t have the bandwidth to do it,” the source says. “That’s the argument for creating these marketplaces, like what a Citi or a FactSet or whoever would be doing, is, in part, they’re providing a vetting service for clients. As to whether that’s enough to get clients using more of this data—I have to believe there have to be some firms out there that are going to want the best data possible to form the best signal, but you have to be trading pretty actively for a lot of it to make sense monetarily.”
The most sensible path forward, rather than competition, may be cooperation. Indeed, early signs of that are beginning to emerge.
Come Together
7Park Data licenses from first-party data sources, taking in their raw data, and transforming those feeds into derived datasets and market indicators. Founded in 2012, the vendor has built an infrastructure that can digest massive, unstructured datasets and turn those into something usable. While that will remain its bread and butter, now it is also essentially licensing that infrastructure out to the sell side.
Brian Lichtenberger, CEO and co-founder of 7Park, compares the service to how Amazon built Web Services to power its e-commerce business, but then realized that the cloud could have utility to others.
“That’s where our services come into play,” he says. “Essentially, we have the ability to repurpose our infrastructure to help data owners get value from their own data. For example, a bank has some type of data on some consumer activity, but it’s not in a form that plugs into their systems or that can be easily analyzed. So they can plug that into our infrastructure in the cloud, we do our work to normalize it and make it useful and drive analytics, then we deliver that data back to them and use that data to make their own decisions internally, or they can take that and commercialize it, or whatever they want to do.”
Certain vendors have said ‘no more data to the sell side,’ because it cannibalizes their own business.
Octavio Marenzi, CEO, Opimas.
He says that “one large bank and another large financial information company” are using the new service. These projects can be as simple as normalizing a bank’s data so that users can click through to receive reports, or more complex use cases, such as creating a panel and normalizing the dataset in a way that both delivers analytics and validates those analytics against other datasets.
“We’ve got real technology that we’ve created over the last six years with a team of experts, engineers and data scientists,” Lichtenberger says. “That’s a highly scalable infrastructure and speaks to a similar end use case that a bank might have with their own data.”
It’s not just the traditional fintech community eyeing this potential new frontier. A year-and-a-half ago, Nasdaq launched its Analytics Hub, the genesis of which came about from its own journey with data science and machine intelligence projects through its Innovation Lab, which was created in 2015.
Through the Analytics Hub, Nasdaq looks to sign exclusive partnerships—such as those with data providers iSentium and Prattle—and bring in other non-exclusive datasets where it structures and cleans the data to provide back to buy-side firms, so they can run their own analytics.
“We’ll never replace the need for our buy-side customers to invest in these capabilities on their own, but our goal is to make it easier for them so they can spend more time on figuring out how to make clean, reliable data work for their processes and investment style, and less time on the data janitor clean-up work,” says Bill Dague, head of alternative data at the exchange.
Dague also believes that Nasdaq “would be great” at helping banks and brokers to monetize their own internal data.
“When you look at the sell-side phenomenon there, in my view when I was out there early on in the Hub days talking to these sell-side banks, I was almost always talking to someone in prime brokerage,” he says. “The idea there is they want to know what’s going on in the market, ‘How can I advise my clients? How can I make sure that I’m up to date on what’s going on?’ What’s interesting is they’re starting to execute and establish a position, but also they’re branching out a little bit and starting to explore the data that they have to offer and monetize that. I think that’s going to be a fraught endeavor in a lot of ways. It will be an interesting development to watch because there’s quite a bit that the banks have to balance. That’s something we could absolutely do; we would be great at it. That falls into our core expertise. I think there’s a lot of opportunity there.”
Over Promise, Under Deliver
Today, there is a certain level of expectation on the buy side when it comes to alternative data. A few years ago, the big race was to find new datasets and stake a claim, not unlike finding and tapping a new well on an oil field, and while that’s still an imperative, by and large, that piece is taken for granted. Now, what is expected is that vendors—and brokers, should they look to enter the space—will provide the analytical tools and visualization to easily extract the value of the raw data, says Octavio Marenzi, CEO of Opimas.
The day that you turn on CNBC and you start to see a segment describing alternate risk premia and the different kinds of factors, that’s the day you know the market is ready.
Head of research at a pension fund.
And if banks want to monetize their own data, it will be more than outside forces pushing back—the call will be coming from inside the house.
“The sell side, in general, has a bit of an antagonistic relationship with the providers of alternative data,” he says. “What the sell side would love to do is use alternative data sources in their equities research and then tell their clients that they analyzed 50 billion credit card transactions and they think this is going on in these sectors, and then the buy side doesn’t need to buy that underlying data service; the sell side will do the heavy lifting for them. [As a result] a lot of the people who sell the data are now saying they’re not going to sell the data to the sell side at all anymore. So certain vendors have said no more data to the sell side because it cannibalizes their own business.”
The head of research at a pension fund with over $30 billion under management is skeptical of these sell-side efforts. In the source’s 20 years on the buy side, they say that they have seen the brokers “offer the world” but routinely under deliver. What often happens is that they understand the market need, but lag when it comes to human resources and the institutional resilience necessary to build robust, interconnected systems and the failure that will eventually underpin any successful technological advancement.
“Before it was about, ‘We’ll help you make better decisions with your stocks,’ now it seems like, ‘We can help you with your data management stuff,’” says the head of research. “The problem with the data management stuff is that there’s an ongoing maintenance element to it that’s really hard to do at arm’s length. So you can have a research service, which is arm’s length—you get an email once or twice a month and here it is and this is what we think about a bunch of stocks to help you do your job. But with the data and technology side, you often need to be much more involved behind the scenes and be much more responsive. It feels like it’s a high value-add, but also a high engagement activity and it’s hard to create scale off of that.”
The source sees the alt-data space for the sell side as being more of a talking point for the brokers when dealing with funds, which—outside of the sophisticated quants—are largely still trying to figure out how they can capitalize on this explosion of new and diverse information.
“The day that you turn on CNBC and you start to see a segment describing alternate risk premia and the different kinds of factors, that’s the day you know the market is ready,” they say. “Until then, you’re going to see earnings announcements and [Jim] Cramer talking about the world—that fairly straightforward, anybody-can-pick-it-up-and-look-at-it language. Until then, much of the alternative data market will remain niche and specialized and not prime to mass distribute.”
Outside of historical problems with bank ownership of data providers, it’s important to recognize that the sell side is also starting to encroach on turf that has been well-tilled already by the vendors of this data, which can be effectively broken down into four categories. There are the first-source providers that actually collect the data to be sold—those that collect the raw satellite imagery or mobile-device pings to cellphone towers. There are the intermediaries that take raw data and transform it into easily-understood metrics. There are the data aggregators and marketplaces that are aiming to become somewhat of an app store or one-stop shop. There’s the already robust consultancy community. All of them have years-long headstarts on new sell-side entrants, and will not be easily dislodged.
Origin Stories
We don’t really do anything for less than three or four years because there’s so much work up front that goes into product development.
Michael Marrale, CEO, M Science.
Founded in 2002, Majestic Research aimed to capitalize on the internet, recognizing early on that people were leaving a digital footprint with every click of their mouse. The vendor would capture that information and sell this early form of alternative data to clients who were hoping to use it to make more informed investment decisions.
In 2010, Majestic was bought by agency broker ITG for $56 million, becoming ITG Investment Research. Then, in 2016, Leucadia National Corporation, the parent of Jefferies Group, bought the vendor and gave it a new name: M Science.
M Science’s early entry into the alt data space was one of fits and starts, but those efforts are now reaping dividends. “I really thought that the buy side would adopt these methodologies, these techniques that we had been utilizing for a decade-and-a-half, much sooner,” says Michael Marrale, the company’s CEO. “What really ended up being an influx of interest, demand and revenue growth for us didn’t really kick in until late 2016, early 2017. Since then, we’ve seen it really pick up.”
In 2019, the company is planning to launch a healthcare practice, as well as expand its industrials coverage. “It’s very early days for us, but if you think about the things that matter to fundamental analysis, similarly we’re exploring ways to using alternative data to gain insight into the entire healthcare ecosystem,” he says. This includes—but is not limited to—pharmaceuticals, biotech, hospitals, payers and providers, where M Science will provide insights into these companies, sectors, and people to help investors in the decision-making process.
But even for a company that has an infrastructure in place to bring in new datasets, what’s involved in the process?
First, just like with oil, there’s exploration. So the process begins with canvassing what’s available—though sometimes, clients will simply come and help M Science to target their efforts around what they specifically want. Once they come across something that they potentially feel could be of value, they must next ensure that the dataset has the proper permissions and use rights in place and that there isn’t any personally identifiable information (PII) available in the data.
M Science has a staff of 115 people—including a 45-person research team—with plans to hire another 40 people in the near future to help with its growth. Those analysts then go through and make a decision on efficacy with regard to alpha generation.
That process, from search to compliance, to testing for a single dataset, can take four-to-six months, Marrale says, and requires members of the tech, data science and research teams to be working in unison. Then, if they actually decide that this is something that should be incorporated into the M Science offering, they will look to sign a multi-year licensing agreement with the provider. “We don’t really do anything for less than three or four years because there’s so much work up front that goes into product development,” he says.
Once they’ve signed the deal, only then do they get to work on turning it into a usable product, by incorporating the dataset into a particular analyst workflow, and, in some cases, creating derived data products for clients.
“What we’re seeing is that those clients that have been working with alternative data over time, as they become more sophisticated they want to go deeper into the data. With every report that we release, we provide backup report analytics and we give our clients the ability to deconstruct our own analysts’ conclusions and take a look at the data for themselves,” Marrale says. “So as clients become more proficient in the use of alternative data, we’re building more data products and data platform solutions that give clients the ability to go and look at the data themselves.”
It’s important to note at this point that even though a bank is looking to monetize its own internal data, the same arduous process described above will likely have to take place. It can be even more complex for banks that have to deal with twitchy legal departments or individual business units not wanting to give the data up—perhaps for reputational risk reasons, perhaps because they’re not inclined to help a competing business unit.
Benefits of Size
M Science is one of many specialist data providers in the market. Its size—as well as smaller companies in the space such as Eagle Alpha, 7Park Data and Quandl—gives it the ability to adjust business plans on the fly. But it must also compete against the prominent data providers in the financial markets, too.
Is an investment bank really going to try to step up and compete with one of those firms? I’d be surprised, but I wouldn’t rule it out.
Emmett Kilduff, CEO, Eagle Alpha
About two years ago, FactSet saw the growth of the alternative data usage on the buy side and tried to figure out how to get into the game. While FactSet was used to integrating data with its own symbology, it had a decision to make: Can it embrace these new and varied datasets, but also consistently tie them into its symbology—otherwise, what’s the value-add in an already populated market? It created the Content & Technology Solutions (CTS) group and appointed Rich Newman to head it, with the goal of combining FactSet’s content along with integrated datasets. “We see our strength as being the data engineers to configure the data and then let our clients run the data science,” Newman says.
Open:FactSet—the vendor’s answer to the buy side’s alt-data needs—is built on top of Microsoft’s Azure cloud infrastructure. In the cloud it has put all of FactSet’s content—its standard feeds, fundamentals, estimates, ownership, supply chain, events, transcripts—alongside partner content, such as datasets from RepRisk and Estimize. “It’s not easy,” Newman says. “I think a lot of people underestimate how hard it is to do the integration.”
The latest offering to be added to the Open:FactSet marketplace is called Data Exploration, which allows users to interact with data from the marketplace in a hosted environment that includes industry-standard databases, programming languages—Python and R—and data visualization tools. Its aim is to allow users to cut down on the time and cost of trialing data internally by doing it in the cloud. The service is being released in a “for-trial environment” currently, Newman says, adding that it will enhance the offering over time so that users can build their production environments directly inside of Open:FactSet.
“You watch a show like Billions and see hedge funds using satellite information, but it’s gone way beyond that now,” he says. “Firms now not only want to look at alternative data like satellite and sentiment but actually data from larger organizations, around weather information and other types of data. We see our obligation as building the platform to enhance that information so that clients can get to the data science more quickly, as opposed to spending all their time doing the integration themselves.”
Similar to the process described by M Science’s Marrale, FactSet has a team of engineers, product developers, strategists and data scientists that are looking through a list of 400-500 potential partners to incorporate into the marketplace. “We won’t put up just any data; there’s a lot of work to incorporate the data, so we want to make sure that there’s client demand,” Newman says. The vendor also has large teams comprising over 1,000 people, located in India and the Philippines, which conduct concordance and matching on the datasets in the marketplace.
Unsurprisingly, the other market data players are already well-established in the space, as well. Take, for example, Bloomberg—you can’t charge over $20,000 for a Terminal license and be seen as being left behind in the alt-data oil rush.
Through the data giant’s Enterprise Data License offering and through Bloomberg Terminal functionality, users can gain access to news and social sentiment analysis, geolocation data, supply chain data, and environmental, social and governance (ESG) datasets.
“We have been providing a variety of alternative datasets through the Terminal for nearly a decade,” says Ben Macdonald, Bloomberg’s global head of enterprise product. “More recently, we have made some of these datasets available through our Enterprise Data License—for example, supply chain, ESG data—and plan to continue expanding our offering, both on the Terminal and through data license.”
And, of course, let’s not forget about the major fund administrators and custodians in the space, such as Northern Trust, who are figuring out how they fit into this new landscape. Peter Sanchez, head of alternative fund services at the custodian bank, says that its job is to have readily-available data for buy-side clients to tap into to enrich their own data warehouse, together with the bank’s data and the fund’s data.
“Five years ago, the idea for them was to wrap controls around the processes and the reports and the services that the administrator was offering to the manager,” Sanchez says. “Now, it’s to the point where, yes, all those controls are in place, but they want to take data management for their oversight and control and transparency to their investors, and so there’s an expectation that you have the means to send data back to the client and they have access to your transaction data, the investor data, the portfolio data, the performance data, in a readily-available way.”
Finding a Spot
Others see themselves falling into the eventual mergers and acquisitions (M&A) shakeup, which is inevitable as the number of alt-data providers reaches a saturation point.
In 2010, Emmett Kilduff started at Morgan Stanley and got an inside look at the bank’s AlphaWise unit, which was created to provide research to the buy side. What he saw inspired him to set up Eagle Alpha six years ago.
Today, the vendor has a database of 850 datasets relevant to the buy side, spread across 24 categories, such as consumer transactions, satellite, social media, and sentiment. It is currently in the process of expanding its oversight to the Asia-Pacific region and, specifically, China.
Eagle Alpha provides data sourcing, dashboards on top of datasets, bespoke alternative data projects, and an industry forum. It’s a self-contained ecosystem, in many ways, and Kilduff says that a deal could be in the future.
“The winner will probably be a type of company that has done that type of job for decades, such as a Bloomberg or FactSet,” he predicts. “They’ve done that for decades, just on traditional data, so why wouldn’t they do it on alternative data? Is an investment bank really going to try to step up and compete with one of those firms? I’d be surprised, but I wouldn’t rule it out. In the end, at Eagle Alpha, we’re humble enough to recognize that we are an early pioneer in the space but we’re more likely to be part of the M&A that will come down the line.”
And still, others are changing the way that they deliver data so as to better position themselves against rivals. Orbital Insight, which has established itself as one of the leading providers of satellite analytics, is going to broaden its offering in 2019. At this year’s Buy-Side Technology North American Summit, held in New York on October 7, Ben Rudin, commercial business lead for Orbital Insight, said the company will eventually use a platform-as-a-service (PaaS) model to deliver information to users.
“This is the future of Orbital Insight,” he said. “Previously, with consumer and energy and a one-off product, that’s currently the model today and in the past, but the future is a platform-as-a-service—[or] as we call it, do-it-yourself geospatial analytics. The end game is to have you, the user, work with our platform to circle anywhere you want in the world or it’s already in our AOI [area of interest] database and you can run your time series yourself to track cars, planes, shipping containers, et cetera—you can do that yourself.”
While Rudin would not get into further specifics of the project, Opimas’ Marenzi, who authored a report examining the alternative data space recently, says that such services are in production already, such as SpaceKnow. He also says this move toward a PaaS offering will help Orbital Insight to move into closer competition with firms such as Quandl, 1010Data, and 7Park Data, “but it will require the company to provide a broader range of datasets and not rely solely on satellite imagery.”
A Whole New World
As the sell side, in general, goes about this transformation, though, it’s worth considering a few key points. Do they have the institutional fortitude to monetize their own internal data? Can they do it better than a tech company that has been doing this kind of thing for years? Can they be better consultants to the buy side even when they, themselves, are trying to figure out how to find lasting signals from this information? And, perhaps most importantly, this: Many of the vendors mentioned above have had to readjust their focus over the years as they’ve learned the pitfalls of the market. Some may succeed, some may fold, and some may be bought. Do siloed banks have that kind of vision, patience, and dexterity to fail and adjust and iterate?
Much like an oil rush, if you get to the game even a shade too late, the well might just have run dry.
Case Study: Citigroup
As was first reported by WatersTechnology’s sibling publication Risk.net, Citigroup is internally figuring out how to best gain value both from its internal data, and how to help buy-side clients capture value from the alt data marketplace at large. It’s a slog, because beyond tech hurdles, there are issues of data confidentiality, breaking down silos in the bank, and making the data useful at scale to justify the effort—without watering down the signals—that need to be overcome.
While Citi declined to comment for both this story and the Risk.net story, a recent job posting on Citi’s website was looking for data scientists to join a new consulting team to help buy-side firms with bespoke data requests covering the alt data field, from finding value in foot traffic metrics, cellphone signals, satellite images, geospatial datasets and transaction data. Citi already provides internal market data to its institutional clients through its Citi Velocity platform at no cost, but this consulting service would help users to navigate the growing quantity of alt data available for consumption.
One portfolio manager at a hedge fund with over $15 billion under management says they were briefed by Citi officials and confirms that they are talking about creating an alt-data specific unit, but that “it didn’t sound like they’re that far ahead when it comes to automating the research.” Rather, that would be left up to the fund itself, with the bank providing support.
The CEO of a niche alt data vendor that includes Citi as a client, was not confident about the current plan as it stands because of competition from the largest data providers, who are—as mentioned previously—already ahead of the curve (at least when compared with the broker community).
“Will Citi’s marketplace work? Probably not. Everyone has a slightly different bend on it. I feel like there’s going to be a long tail but with a few people that nail it, but it will probably be a Bloomberg or FactSet—someone like that,” says the source. “These guys are really trying hard to sign exclusives on these things so right now everyone is just taking sides.”
Earlier this year, Citi began to give clients access to Thinknum’s analytics. Much like M Science, Thinknum was originally created to take advantage of the internet by creating a platform where people could share their analysis of companies, share financial models, and share web tools to allow investors to be more efficient. But when the company went to market, they realized the bigger pain point was getting access to data, says Justin Zhen, co-founder of Thinknum. While this may fly in the face of the analytics imperative mentioned before, their focus is on web crawling, which is the Wild West, in many ways, as the internet has been described as the ultimate dataset.
Thinknum has built a data index around this information and connects how these sources of information from myriad websites can drive a signal. They offer insights into 30 datasets, but one dataset can cover as many as 400,000 companies, Zhen says. They crawl data from third-party websites and then organize and structure that information.
Zhen declined to talk about Thinknum’s partnership with Citi, but said that this type of work, for buy side firms, follows a familiar pattern in that it allows them to focus on what they’re good at—trading—rather than being distracted by data work.
“They [hedge funds wanting to incorporate this information] want to spend their time analyzing the data; they don’t want to collect the data, clean it and organize it,” Zhen says. “They validate [their information] from us and then spend time doing analysis on it and trading off of it.”
While Citi—and every other bank in the space—will face a tough road ahead, if they do figure out how to crack the alt data market open, there are riches to be gained, says Opimas’ Marenzi, who was formerly the CEO of consultancy Celent and worked in UBS’s asset management business division in Zurich previously.
“In terms of Citibank doing this, they’ve been talking about doing something in this space for a long time and I haven’t talked to them for a few months, but my sense is that they’re still in the exploratory phase with this and are having a tough time bringing something to market,” he says. “But the advantage that someone like Citibank could have is they could have some very unique datasets that other people don’t have. So Citibank—or other large commercial banks—could provide huge insights into payment movements, cross-border payments, they can probably see a large part of the US payroll numbers before anyone else does, they can see creditworthiness and credit quality, they can probably tell you what’s going on in the commercial mortgage space and, by extrapolation, commercial real estate. They would have a huge amount of data internally—if they can get permission to access that it would be tremendously valuable.”
Only users who have a paid subscription or are part of a corporate subscription are able to print or copy content.
To access these options, along with all other subscription benefits, please contact info@waterstechnology.com or view our subscription options here: http://subscriptions.waterstechnology.com/subscribe
You are currently unable to print this content. Please contact info@waterstechnology.com to find out more.
You are currently unable to copy this content. Please contact info@waterstechnology.com to find out more.
Copyright Infopro Digital Limited. All rights reserved.
You may share this content using our article tools. Printing this content is for the sole use of the Authorised User (named subscriber), as outlined in our terms and conditions - https://www.infopro-insight.com/terms-conditions/insight-subscriptions/
If you would like to purchase additional rights please email info@waterstechnology.com
Copyright Infopro Digital Limited. All rights reserved.
You may share this content using our article tools. Copying this content is for the sole use of the Authorised User (named subscriber), as outlined in our terms and conditions - https://www.infopro-insight.com/terms-conditions/insight-subscriptions/
If you would like to purchase additional rights please email info@waterstechnology.com
More on Regulation
Verafin launches genAI copilot for fincrime investigators
Features include document summarization and improved research tools.
Waters Wrap: Open source and storm clouds on the horizon
Regulators and politicians in America and Europe are increasingly concerned about AI—and, by extension, open-source development. Anthony says there are real reasons for concern.
DSB says industry is ready to meet UPI mandate ahead of deadline
The Unique Product Identifier will be required for certain OTC derivatives in the EU at the end of April, following US adoption in January.
‘Very careful thought’: T+1 will introduce costs, complexities for ETF traders
When the US moves to T+1 at the end of May 2024, firms trading ETFs will need to automate their workflows as much as possible to avoid "settlement misalignment" and additional costs.
Court case probes open-source licenses as movement stands at crossroads
The Software Freedom Conservancy’s lawsuit against TV-maker Vizio begins trial in California, raising questions about open-source licenses and the risks posed by adhering to them.
Waters Wavelength Podcast: Countdown to T+1
DTCC’s Val Wotton joins the podcast this week to discuss the impending move to T+1 in the US.
Consolidated tape hopefuls gear up for uncertain tender process
The bond tapes in the UK and EU are on track to be authorized in 2025. Prospective bidders for the role of provider must choose where to focus their efforts in anticipation of more regulatory clarity on the tender process.
Fighting FAIRR: Inside the bill aiming to keep AI and algos honest
The Financial Artificial Intelligence Risk Reduction Act seeks to fix a market abuse loophole by declaring that AI algorithms do not have brains.
Most read
- Chris Edmonds takes the reins at ICE Fixed Income and Data Services
- Deutsche Börse democratizes data with Marketplace offering
- Nasdaq reshuffles tech divisions post-Adenza