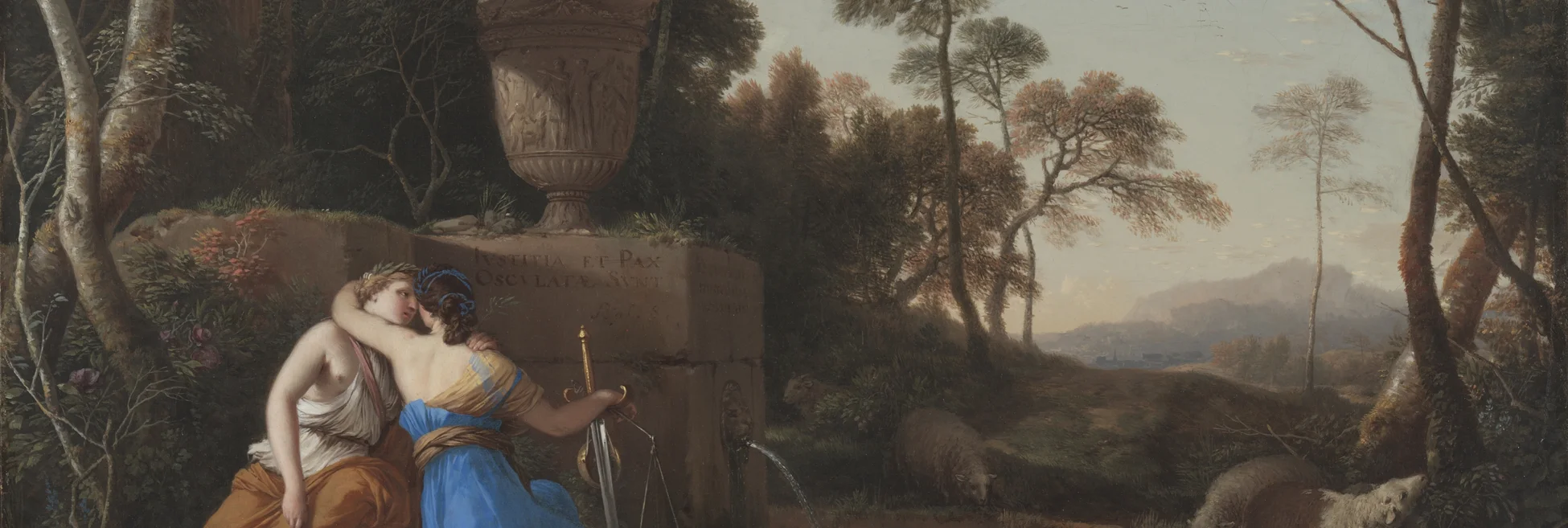
Waters Wrap: Unintended Consequences & AI Regulation (And Mobile Trading Reg & BERT NLP)
Due to the pandemic and rapid advancements in the fields of AI and mobile technology, regulators in the US and Europe have unique challenges on their hands.
This week, we looked at how regulators in Europe are exploring how best to oversee AI development in the capital markets (and the potential unintended consequences if they aren’t careful while writing these rules). We also look at how US regulators are considering being more prescriptive in regulating remote trading, and might decide to match similar requirements in Europe. And, finally, we talk about the use of natural language processing in capital markets and how Google’s transformer-based model BERT is a game-changer for NLP’s growth.
Lots to unpack here, so let’s get to it.
That Old Law of Unintended Consequences
Macquarie Island sits about halfway between Australia and Antarctica. After it was discovered at the turn on the 19th century, it became a hotbed for seal and penguin hunters, as well as explorers. The ships bringing the hunters and explorers introduced rats and mice to the island, the populations of which grew quickly as there weren’t any natural predators around. So they brought one: cats. And after the kitties rose up the island’s food chain, another newcomer arrived in the late 1800s—rabbits for both the cats and hunters to feast on.
There’s a bawdy old saying about how much rabbits like to have intercourse, and since rabbits are completely irresponsible and don’t use protection, they breed quickly. To curtail the following baby boom, scientists introduced a rabbit flea to curb the rabbit population. But, of course, that created a new problem. As the cats needed to find a new source of food, they turned their collective attention to seabirds, and so then conservationists needed a cat eradication plan.
Not unsurprisingly, no cats meant that the bunnies who weren’t killed by the flea could start repopulating—and repopulate, they did, eating huge swaths of the island’s vegetation, which caused “exotic grasses and herbs [to take over] the naked slopes, forming a dense network of leaves and stems that, in some places, prevented native seabirds from accessing suitable nesting sites.” Conservationists say it will cost A$24 million (~$17 million) to restore the island.
This is one of my favorite examples of the law of unintended consequences and I was reminded of it while reading Jo Wright’s opinion piece on the European Commission’s attempt to regulate artificial intelligence. Most notably, the EC’s early statements on future regulations could lay blame at the feet of the chief technology officer—or chief information officer, or head of technology—overseeing an AI project, should a catastrophic glitch occur as a result of the AI’s coding.
“A major aspect of this approach, as set out in the whitepaper, is adapting existing EU liability concepts to AI,” Jo writes. “Firstly, ‘strict liability’ must lie with the person who is in control of the risk associated with the operation of the AI. Strict liability means that the producer of an AI product is liable for harm resulting from its use, even if they were ignorant of the fault in the product. These operators also have duties of care, including monitoring the system, the report says.”
John Ahern, a partner in the financial services group at law firm Covington in London, said that if the EC isn’t careful in writing future AI legislation, that old law of unintended consequences could kick in.
“What we need to be attentive to is where in the chain of liability are individuals affected,” he told Jo. “Think, for example, of the CTO: if there is a flaw in the design of a database product, or an algo, or any other form of AI product or service, does that mean that, from either a liability or a regulatory perspective, the human being overseeing the process or the product design or the development now has an increased risk of liability in one form or another? … And where individuals have regulatory or legal liability, there is a risk/reward quotient that comes into taking on that role, and the risk increases.”
I was reading a story on TechCrunch about Albion College in Michigan and how it was going to “require students to download and install a contact-tracing app called Aura, which it says will help it tackle any coronavirus outbreak on campus. There’s a catch. The app is designed to track students’ real-time locations around the clock, and there is no way to opt out. … Worse, the app had at least two security vulnerabilities only discovered after the app was rolled out. One of the vulnerabilities allowed access to the app’s back-end servers. The other allowed us to infer a student’s Covid-19 test results.”
Technology is slowly eroding our privacy and there’s a tipping point coming: with a global pandemic (or in the name of thwarting terrorist activities, or reducing gun violence in a city, or catching an individual that defiles government property) how much are the government and institutions—both public and private—allowed to monitor citizens in the name of the public good?
And it’s not only Big Brother who is diminishing our privacy: the other day, a drone flew right outside the living room window of my apartment—am I not entitled to privacy in my own living room? Or, much more sinisterly, what happens in the case that a man flies a drone outside the window of his ex-girlfriend or ex-wife, even though she has an active restraining order against him?
Those are the questions that I want regulators addressing, and addressing responsibly. But they must be careful regulating the technology itself. Drone technology is used for an assortment of good uses, from conservation efforts and anti-poaching enforcement, to agriculture monitoring and health care delivery. There are also very valid uses of contact-tracing apps—you don’t regulate that technology, you regulate how that data is used and overseen.
When Knight Capital collapsed after a computer glitch led to a $400 million loss, the company had to be sold to Getco and many people lost their jobs.
Now we can have a different conversation about golden parachutes and the actual misdeeds of bankers that led to the Financial Crisis, but did Steven Sadoff, who headed Knight’s tech unit at the time of the crash, deserve to be brought before a magistrate? Absolutely not. Mistakes were made and very painful lessons learned, but there wasn’t anything malicious built into the coding of the firm’s trading platform.
Do I think that the EC is purposely setting its sights on the heads of technology? No, no I do not. But just because that is not their intent, doesn’t mean a clever lawyer can’t strongly make that case if the Commission isn’t careful in wording its future AI regulations.
Need a Prescription?
Last week, Luke Clancy wrote about how banks are putting innovative, “moonshot” projects on the backburner in favor of prioritizing remote-working tools. And as we’ve previously reported, banks are likely to continue to turn to virtual desktops, surveillance tech, outsourced-trading solutions, and the continued adoption of natural language processing (NLP) and use of open-source tools in a post-Covid-19 world, but away from virtual private networks (VPNs) and—hopefully—systems that are programmed in Cobol.
But one thing that has become clear—at least in the US—is that trading firms have been left largely up to their own devices when incorporating these remote-work strategies. When it comes to regulation in the capital markets, it’s a delicate balance between adhering to certain principals of free and open markets, and the need to be prescriptive when the markets and firms can’t—or shouldn’t—regulate themselves. In the US, it would appear as though many firms were winging it when it came to enacting fully-remote trading environments.
As Reb Natale reports, the Securities and Exchange Commission’s Office of Compliance Inspections and Examinations (OCIE) has observed areas of heightened risk that it says firms should be focusing on during the pandemic. Some regulatory experts think that the coronavirus will lead US regulators to reevaluate how prescriptive they are when writing rules around remote trading and alternative devices, like personal laptops and cell phones.
Danielle Tierney, a senior adviser on market structure and technology at Greenwich Associates, tells Reb that the SEC may be laying the groundwork to enact more specific regulation with exact procedures that more closely align with the EU’s Market Abuse Regulation (MAR) and the Markets in Financial Instruments Directive (Mifid II). For example, US regulation on mobile phones used in trading is vague, at best, whereas MAR and Mifid II directly address those devices in their communications monitoring requirements.
“It’s been up to firms to self-regulate. It’s been up to firms to say, ‘Okay, it’s a pain to have all this mobile surveillance functionality, and roll out all these devices, and it costs money.’ But also, if we don’t monitor any of these mobile devices that employees are definitely using for work, then they’re basically just whistling past the graveyard until someone does something really, really bad,” Tierney said.
There are several other issues that that article explores around surveillance, cybersecurity, and regulation, but this is an area that I think that even most free-market individuals would agree that regulators will need to take on a bigger role. Again, you don’t ban the cutting-edge technology that is allowing for ever-increasing mobility; you regulate the market participants to ensure that everyone is trading in a fair and just environment.
It kind of reminds me of baseball, actually. In the mid-1980s on through the 2000s, the science around performance-enhancing drugs (PEDs) advanced exponentially and seeped into professional sports. Major League Baseball (MLB) made the conscious decision not to enact mandatory drug testing until after many of baseball’s hallowed records were shattered.
You can’t blame the science (or the technology, if you will)—steroids and human growth hormone (HGH) are incredibly-helpful drugs prescribed legally by doctors. You can’t really blame the ballplayers, because while maybe not everyone was on some sort of drug in the mid-80s into the early 2000s, it’s clear that most were taking at least something at some point in their careers. Who was it really hurting, and both the fans and management were happy with the results. (For what it’s worth, I think PEDs should be legal—let me see what kind of athletes science can create. This is entertainment—as long as everyone knows the risks…play ball!)
No, it was MLB’s fault for not properly creating a level playing field where everyone knew clearly what the rules were and how those rules would be enforced. And this is something that MLB ran into a few years later when, once again, MLB turned a blind eye to sign stealing before technology allowed the practice to get out of control.
If the SEC isn’t a bit more proactive and prescriptive in monitoring remote trading, well then don’t be surprised when traders and senior managers go tip-toeing up to unwritten lines and decide to continue to walk on past those imaginary—or at least gray—lines.
Hey, BERT!
Wei-Shen Wong reported this week that IHS Markit is using Google’s transformer-based model BERT and a combination of classification and extraction techniques to determine what the vendor’s internal documents mean and then summarize them.
By the end of Q4, the data service provider aims to upload about one million documents published by internal analysts over the past 10 years. The research reports cover topics related to financial services, the automotive industry, agriculture, chemicals, economics and country risks, energy, life sciences, and more.
Now BERT stands for Bidirectional Encoder Representations from Transformers, which is something that I don’t think I could cleanly say totally sober, but the model is proving to be a game-changer in the field of NLP and for capital markets firms.
Back at the beginning of March, Jo Wright wrote a couple-thousand words about how BERT, which Google released in 2018, is gaining traction at companies like Refinitiv and Bloomberg. When it was rolled out, the open-sourced model had already been pre-trained on Wikipedia and a dataset called BookCorpus, which includes about 3 million words. Because all that pre-training heavy lifting was already done, financial services firms simply needed to fine tune the model using terms specific to the world of trading.
BERT’s underpinning transformer model and deep neural networks are not new, but the fact that it’s been open-sourced and it is relatively easy to use is allowing an array of researchers to experiment with it and development cutting-edge use cases. (And to be sure, BERT is not the only transformer model in the field—there’s also ELMo and GPT-2, which were trained in different ways, but the basic architecture is the same.)
So, for an example as to how it’s being used in the capital markets, Refinitiv took BERT’s pre-trained dictionary and added its library of Reuters news stories to create a robust “dictionary,” though it required a lot of pre-processing and training time to get right, Tim Nugent, senior research scientist at Refinitiv, told Jo. One of the first areas they applied the model to was ESG.
“ESG is a hot topic; it’s a nice place to start,” Nugent said. “We have a lot of ESG data; a lot of it is annotated by our many analysts; we deem it to be high-quality; it has had a lot of human input. Analysts have scrutinized and classified it, and the specific controversy dataset into one of about 20 different ESG controversy types—things like privacy and environmental controversies. … We ran this using standard BERT, and then we ran it using our domain-specific version of BERT. We didn’t worry too much about the absolute level of perfection—we were looking for the relative level of improvement between BERT and the domain-specific version of BERT.”
You can read the article for more on Refinitiv’s ESG project, as well as how Bloomberg is using BERT to improve the Terminal experience for traders. And, of course, you can click here to read about IHS Markit’s experience using BERT on its own data.
What I find most interesting, though, is that just three years ago, most of the talk in the capital markets was about using machine-learning models and algorithms, but now I get the feeling that it is NLP that’s getting more investment. Obviously, there’s overlap between ML and NLP, but it does seem that as more advancements are made in the field of open-sourced NLP tools, companies are finding more success using these models on their years of data, rather than trying to build some cutting-edge, deep-learning model.
Or, maybe because much of the advancements are being open-sourced, firms are just more willing to talk about their NLP endeavors rather than talk openly about those mysterious black-box machine-learning models.
It’s a discussion for another time. There’s more I wanted to talk about, but we’re well over 2,000 words. See you next Sunday.
Further reading
Only users who have a paid subscription or are part of a corporate subscription are able to print or copy content.
To access these options, along with all other subscription benefits, please contact info@waterstechnology.com or view our subscription options here: http://subscriptions.waterstechnology.com/subscribe
You are currently unable to print this content. Please contact info@waterstechnology.com to find out more.
You are currently unable to copy this content. Please contact info@waterstechnology.com to find out more.
Copyright Infopro Digital Limited. All rights reserved.
You may share this content using our article tools. Printing this content is for the sole use of the Authorised User (named subscriber), as outlined in our terms and conditions - https://www.infopro-insight.com/terms-conditions/insight-subscriptions/
If you would like to purchase additional rights please email info@waterstechnology.com
Copyright Infopro Digital Limited. All rights reserved.
You may share this content using our article tools. Copying this content is for the sole use of the Authorised User (named subscriber), as outlined in our terms and conditions - https://www.infopro-insight.com/terms-conditions/insight-subscriptions/
If you would like to purchase additional rights please email info@waterstechnology.com
More on Regulation
Verafin launches genAI copilot for fincrime investigators
Features include document summarization and improved research tools.
Waters Wrap: Open source and storm clouds on the horizon
Regulators and politicians in America and Europe are increasingly concerned about AI—and, by extension, open-source development. Anthony says there are real reasons for concern.
DSB says industry is ready to meet UPI mandate ahead of deadline
The Unique Product Identifier will be required for certain OTC derivatives in the EU at the end of April, following US adoption in January.
‘Very careful thought’: T+1 will introduce costs, complexities for ETF traders
When the US moves to T+1 at the end of May 2024, firms trading ETFs will need to automate their workflows as much as possible to avoid "settlement misalignment" and additional costs.
Court case probes open-source licenses as movement stands at crossroads
The Software Freedom Conservancy’s lawsuit against TV-maker Vizio begins trial in California, raising questions about open-source licenses and the risks posed by adhering to them.
Waters Wavelength Podcast: Countdown to T+1
DTCC’s Val Wotton joins the podcast this week to discuss the impending move to T+1 in the US.
Consolidated tape hopefuls gear up for uncertain tender process
The bond tapes in the UK and EU are on track to be authorized in 2025. Prospective bidders for the role of provider must choose where to focus their efforts in anticipation of more regulatory clarity on the tender process.
Fighting FAIRR: Inside the bill aiming to keep AI and algos honest
The Financial Artificial Intelligence Risk Reduction Act seeks to fix a market abuse loophole by declaring that AI algorithms do not have brains.
Most read
- Chris Edmonds takes the reins at ICE Fixed Income and Data Services
- Deutsche Börse democratizes data with Marketplace offering
- Nasdaq reshuffles tech divisions post-Adenza