Cutting Big Data Down to Size
Editor's View
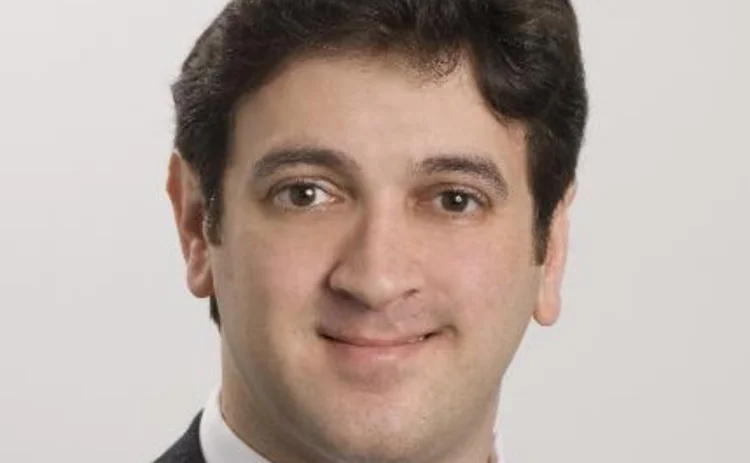
Last week, I wrote about a choice that could need to be made between cloud resources and the Hadoop tool for managing and working with big data. A better question, or a better way to frame the debate, could be as a decision about what is the best way to make use of cloud computing for data management, especially for "big data."
Tim Vogel, a veteran of data management projects for several major investment firms and service providers on Wall Street, has focused views on this subject. He advises that the cloud is best used for the most immediate real-time data and analytics. As an example, Vogel says the cloud would be an appropriate resource if one was concerned with the most recent five minutes of data under volume-weighted average pricing (VWAP).
"The cloud isn't cheap," he says. "Its best use is not for data on a security that hasn't traded in two weeks. Unless the objective is to cover the complete global universe, like [agency broker and trading technology provider] ITG does." Vogel points to intra-day pricing and intra-day analytics as tasks that could be enhanced, accelerated or otherwise improved upon through use of cloud computing resources. Data managers should think of securities data in two layers—a descriptive or identification layer and a pricing layer—both of which have to be processed and filtered to generate usable data that goes into cloud resources.
The active universe of securities as a whole, which includes fundamental data and analytics on securities, is really a super-set of what firms are trying to handle in terms of data on a daily basis, observes Vogel. With that in mind, the task for applying cloud computing to big data could actually be making big data smaller, or breaking it down into parts—cutting it down to size. That certainly will cut down on the bandwidth needed to send and retrieve data to and from the cloud, and consistently reconcile local data and cloud-stored data.
If nothing else, this is certainly a different way of looking at handling big data. It is worth considering whether going against the conventional or prevailing wisdom could lead data managers to a better way. Inside Reference Data would like to know what you think about this. We've reactivated our LinkedIn discussion group, where you can keep up with new stories being posted online, live tweets covering conference discussions, and provide feedback to questions and opinion pieces from IRD.
Only users who have a paid subscription or are part of a corporate subscription are able to print or copy content.
To access these options, along with all other subscription benefits, please contact info@waterstechnology.com or view our subscription options here: https://subscriptions.waterstechnology.com/subscribe
You are currently unable to print this content. Please contact info@waterstechnology.com to find out more.
You are currently unable to copy this content. Please contact info@waterstechnology.com to find out more.
Copyright Infopro Digital Limited. All rights reserved.
As outlined in our terms and conditions, https://www.infopro-digital.com/terms-and-conditions/subscriptions/ (point 2.4), printing is limited to a single copy.
If you would like to purchase additional rights please email info@waterstechnology.com
Copyright Infopro Digital Limited. All rights reserved.
You may share this content using our article tools. As outlined in our terms and conditions, https://www.infopro-digital.com/terms-and-conditions/subscriptions/ (clause 2.4), an Authorised User may only make one copy of the materials for their own personal use. You must also comply with the restrictions in clause 2.5.
If you would like to purchase additional rights please email info@waterstechnology.com
More on Data Management
EDM Council expands reach with Object Management Group merger
The rebranded EDM Council now includes members from industries outside financial services.
As datacenter cooling issues rise, FPGAs could help
IMD Wrap: As temperatures are spiking, so too is demand for capacity related to AI applications. Max says FPGAs could help to ease the burden being forced on datacenters.
Bloomberg introduces geopolitical country-of-risk scores to terminal
Through a new partnership with Seerist, terminal users can now access risk data on seven million companies and 245 countries.
A network of Cusip workarounds keeps the retirement industry humming
Restrictive data licenses—the subject of an ongoing antitrust case against Cusip Global Services—are felt keenly in the retirement space, where an amalgam of identifiers meant to ensure licensing compliance create headaches for investment advisers and investors.
LLMs are making alternative datasets ‘fuzzy’
Waters Wrap: While large language models and generative/agentic AI offer an endless amount of opportunity, they are also exposing unforeseen risks and challenges.
Cloud offers promise for execs struggling with legacy tech
Tech execs from the buy side and vendor world are still grappling with how to handle legacy technology and where the cloud should step in.
Bloomberg expands user access to new AI document search tool
An evolution of previous AI-enabled features, the new capability allows users to search terminal content as well as their firm’s proprietary content by asking natural language questions.
CDOs must deliver short-term wins ‘that people give a crap about’
The IMD Wrap: Why bother having a CDO when so many firms replace them so often? Some say CDOs should stop focusing on perfection, and focus instead on immediate deliverables that demonstrate value to the broader business.