The Proper Characteristics of Data Scientists and How to Use Them
Why domain knowledge matters when it comes to firms' data scientists.
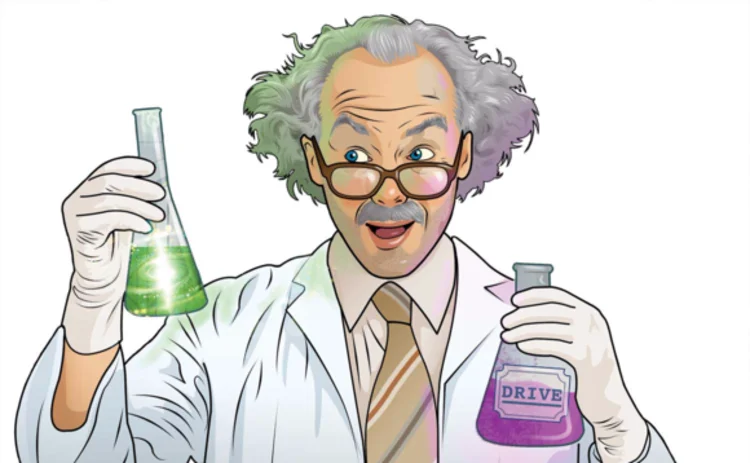
There is nothing quite like a good debate between two panelists at a conference.
Our conference producers do a great job of assembling some of the best and brightest in financial technology to speak on a variety of subjects, and whenever we can get a few of them to go back and forth on a topic they disagree on, it usually leads to some great insight.
That was the case last week on an algorithms and analytics panel at the North American Trading Architecture Summit in New York. A few of the panelists discussed the merits of whether or not it was critical for data scientists to have domain knowledge.
I've already written about the panel, but to give you a brief summary of how it all went down: Gary Bhattacharjee of Morgan Stanley Wealth Management said data scientists should have domain knowledge; Ron Papka, formerly of Citi, disagreed, saying sometimes he feels it's better if a data scientist doesn't have any domain knowledge.
Know the Business
I'll admit, after hearing the two go back and forth, I tend to side with Bhattacharjee. His anecdote about data scientists coming up with a hypothesis that the firm should promote everyone to managing director was a perfect example of why everyone, even those in technology, should have at least a slight understanding of the business they're solving problems for.
I've written about this before, but I've had the opportunity to interview several CTOs and CIOs about their career paths. Not all of them had master's degrees in business administration, but they did all spend a period of their careers learning the financial markets, whether it was spending time in a specific vertical or going back to school.
I even posed the question to a CTO at a technology vendor I met with Tuesday: How important is domain knowledge to a data scientist? He sided with Bhattacharjee, citing a similar anecdote about data scientists who didn't understand the markets coming up with a solution for a problem that was in no way practical.
That's not to say I don't see validity in what Papka said. I do believe bringing in a person with a fresh perspective that isn't biased toward certain processes is helpful. More often than not, it's a fresh set of eyes that hasn't been tainted by traditional methods that can be the most innovative.
However, I think you always need at least someone on staff with that domain knowledge who has the ability to make sure the solution is practical.
Getting Work Done
One part of the panel I did find interesting that I wasn't able to include in my story was Papka's criticism of the lack of actual successful use cases of data scientists on Wall Street.
"People are investing in it and they haven't really shown benefits from it," Papka said. "Data science is a buzzword. A lot of people don't know what it actually means, but they really believe that this is the future. It's very hard to find an algorithm that actually helps the business, a predictive model that actually leads to something actionable. So you could find a correlation, but it doesn't mean that you could use it for your business purposes."
In Papka's opinion, there are only two formulas for the successful use of data scientists. The first is what he called a unicorn: a one-person wrecking ball who reports to the top of the business and has access to all the data. The other is a team effort that consists of a group of scientists that have gone through a formal science education.
Papka admitted the two approaches are on opposite ends of the spectrum, but said it's the only two ways he's found data scientists to be successful.
The unicorn approach seems particularly difficult, in my opinion. To find one person with that type of range and management skills would be a big ask, which Papka admitted. Also, I'm not sure how successful a single person could be at a massive asset manager or bank.
Still, I do agree with Papka that some type of hybrid approach might not necessarily be successful. Too often, firms try and hedge their bets by not fully committing to a technology. More often than not that only leads to failed projects and lost capital.
This week on the Waters Wavelength podcast ─ Episode 14: NATAS, Sell-Side Technology Awards
If you haven't already, subscribe to the podcast on iTunes here. Also, check out our SoundCloud account here.
Food for Thought
- I'm heading to Miami Beach for Sifma Ops 2016. I'll be arriving Monday and leaving Thursday. If you'll be down there and would like to meet up, drop me a line here.
- The Sell-Side Technology Awards were announced on Thursday. Congratulations to all the winners. You can find the complete list of winners here. In the coming weeks, we'll release individual write-ups on all the winners.
- "Game of Thrones" has gotten all the hype this week, but for the technology folks, I truly suggest "Silicon Valley." I know I'm not breaking any news here, but it's an absolutely fantastic show and worth checking out for those of you who haven't gotten around to it yet.
Only users who have a paid subscription or are part of a corporate subscription are able to print or copy content.
To access these options, along with all other subscription benefits, please contact info@waterstechnology.com or view our subscription options here: https://subscriptions.waterstechnology.com/subscribe
You are currently unable to print this content. Please contact info@waterstechnology.com to find out more.
You are currently unable to copy this content. Please contact info@waterstechnology.com to find out more.
Copyright Infopro Digital Limited. All rights reserved.
As outlined in our terms and conditions, https://www.infopro-digital.com/terms-and-conditions/subscriptions/ (point 2.4), printing is limited to a single copy.
If you would like to purchase additional rights please email info@waterstechnology.com
Copyright Infopro Digital Limited. All rights reserved.
You may share this content using our article tools. As outlined in our terms and conditions, https://www.infopro-digital.com/terms-and-conditions/subscriptions/ (clause 2.4), an Authorised User may only make one copy of the materials for their own personal use. You must also comply with the restrictions in clause 2.5.
If you would like to purchase additional rights please email info@waterstechnology.com
More on Emerging Technologies
Waters Wavelength Ep. 324: A philosophical conversation about AI
This week, Reb and Nyela discuss BNY’s digital workers, and what the use of AI in society signals for the future.
Cloud Wars: Are EU and APAC firms really pining for homegrown options?
Waters Wrap: In the wake of tariffs and regional instability, there’s chatter about non-US firms lessening their dependency on the major hyperscalers. Anthony is not buying it.
Google gifts Linux, capital raised for Canton, one less CTP bid, and more
The Waters Cooler: Banks team up for open-source AI controls, S&P injects GenAI into Capital IQ, and Goldman Sachs employees get their own AI assistant in this week’s news roundup.
Numerix strikes Hundsun deal as China pushes domestic tech
The homegrown tech initiative—‘Xinchuang’—is a new challenge for foreign vendors.
RBC’s partnership with GenAI vendor Cohere begins to bear fruit
The platform aims to help the Canadian bank achieve its lofty AI goals.
Deutsche Bank casts a cautious eye towards agentic AI
“An AI worker is something that is really buildable,” says innovation and AI head
TMX buys ETF biz, Iress reinvests in trading tools, UBS data exposed, and more
The Waters Cooler: Euroclear’s next-gen service, MarketAxess launches e-trading for IGBs, and new FX services are in this week’s news round-up.
SEC pulls rulemaking proposals in bid for course correction
The regulator withdrew 14 Gensler-era proposals, including the controversial predictive data analytics proposal.