AFTAs 2017: Most Cutting-Edge IT Initiative—JPMorgan Asset & Wealth Management
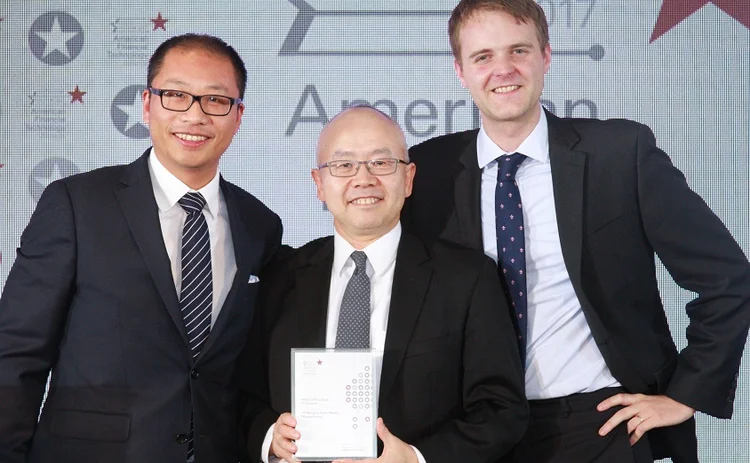
“The latest instantiation took approximately three months to develop and was built through strong partnership across the investment team and the technology team, with two members of the investment team with PhDs in artificial intelligence, and three technologists—two focused on the server side, and one focused on the graphical user interface front-end—focused on the project,” says Kent Zheng, head of global research technology for EMEA at JPMorgan Asset Management. The tool sifts through hundreds of news sources received through providers including Bloomberg and FactSet, along with wider unstructured data each day, using a recurrent neural network that is continuously trained by manually labelled data to pick up patterns that distinguish M&A-related news articles from others. “The NewsFilter picks up words from unstructured news articles in free text format and filters out uninformative components, forming a domain-specific vocabulary and transforming the input into a structured form. We use a distributed representation to translate the words in the text to predictive features. This allows semantically similar words to cluster in a high-dimensional space. In addition, we initialize our deep-learning process with pre-trained vector embeddings to leverage the rich semantic domain knowledge captured by large studies carried out on billions of news items and Wikipedia articles,” says Zheng.
The tool is useful in removing potential sources of “idiosyncratic risk,” says Yazann Romahi, chief investment officer of QBS at the firm. This is useful in the rumor-mired world of M&A. Romahi estimates that the machine-learning enhancements have improved performance in long/short by up to 0.5 percent per year, by filtering out and avoiding up to eight potential shorts per year. NewsFilter is under continuous development. Along with the ongoing training of the neural network, it also takes into account “user feedback to dynamically improve its predictions,” Zheng says. “This is a fast-moving field, and we regularly carry out model comparisons to incorporate the newest methodologies into our solution. On top of relevance, we are also expanding the classification framework to be able to distinguish between actionable news items versus rumors. We are also looking to develop a novelty factor component that can verify whether a news article contains new deal information that was not captured by articles that preceded it,” he says.
Only users who have a paid subscription or are part of a corporate subscription are able to print or copy content.
To access these options, along with all other subscription benefits, please contact info@waterstechnology.com or view our subscription options here: https://subscriptions.waterstechnology.com/subscribe
You are currently unable to print this content. Please contact info@waterstechnology.com to find out more.
You are currently unable to copy this content. Please contact info@waterstechnology.com to find out more.
Copyright Infopro Digital Limited. All rights reserved.
As outlined in our terms and conditions, https://www.infopro-digital.com/terms-and-conditions/subscriptions/ (point 2.4), printing is limited to a single copy.
If you would like to purchase additional rights please email info@waterstechnology.com
Copyright Infopro Digital Limited. All rights reserved.
You may share this content using our article tools. As outlined in our terms and conditions, https://www.infopro-digital.com/terms-and-conditions/subscriptions/ (clause 2.4), an Authorised User may only make one copy of the materials for their own personal use. You must also comply with the restrictions in clause 2.5.
If you would like to purchase additional rights please email info@waterstechnology.com
More on Awards & Rankings
Best data governance solution—Siepe
Siepe wins Best data governance solution at the IMD & IRD Awards 2025.
Best reference data initiative—Derivatives Services Bureau
The DSB wins Best reference data initiative at the IMD & IRD Awards 2025.
Best corporate actions managed service—S&P Global Market Intelligence
S&P Global Market Intelligence wins Best corporate actions managed service at the IMD & IRD Awards 2025.
Sell-Side Technology Awards 2025: Best distributed-ledger technology—Broadridge Financial Solutions
Project: Distributed Ledger Repo (DLR)
Sell-Side Technology Awards 2025: Best sell-side back-office platform—FIS
Product: FIS Post Trade Processing Platform