AML models face explainability challenges
Data gaps and potential biases must be accounted for in approaches to tackling money laundering.
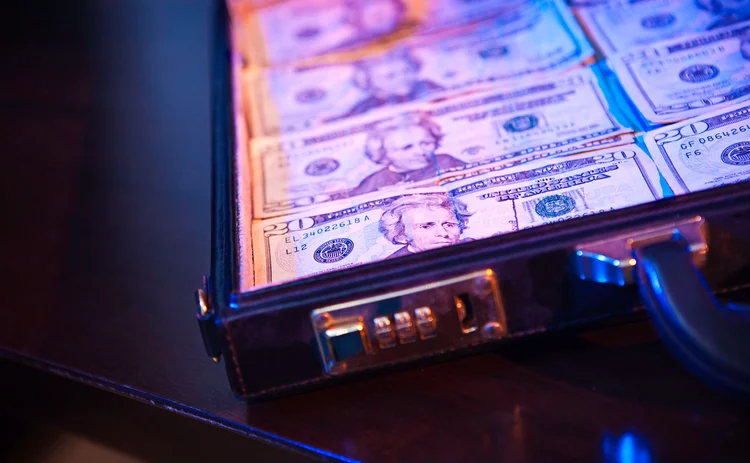
Two years after the US Federal Reserve gave its blessing to banks to pursue artificial intelligence-led approaches to combat financial crime, lenders fear the pendulum might be about to swing back the other way.
Banks have found great early success in piloting machine-learning techniques to spot suspicious transactions and identify weaknesses in existing controls, with their power to divine patterns in disparate datasets making them more effective than legacy rules-based systems that find too
Only users who have a paid subscription or are part of a corporate subscription are able to print or copy content.
To access these options, along with all other subscription benefits, please contact info@waterstechnology.com or view our subscription options here: http://subscriptions.waterstechnology.com/subscribe
You are currently unable to print this content. Please contact info@waterstechnology.com to find out more.
You are currently unable to copy this content. Please contact info@waterstechnology.com to find out more.
Copyright Infopro Digital Limited. All rights reserved.
As outlined in our terms and conditions, https://www.infopro-digital.com/terms-and-conditions/subscriptions/ (point 2.4), printing is limited to a single copy.
If you would like to purchase additional rights please email info@waterstechnology.com
Copyright Infopro Digital Limited. All rights reserved.
You may share this content using our article tools. As outlined in our terms and conditions, https://www.infopro-digital.com/terms-and-conditions/subscriptions/ (clause 2.4), an Authorised User may only make one copy of the materials for their own personal use. You must also comply with the restrictions in clause 2.5.
If you would like to purchase additional rights please email info@waterstechnology.com
More on Emerging Technologies
M&A activity, syndicated loans, a new tariff tool, and more
The Waters Cooler: LSEG and LeveL Markets partner for new order type, QuantHouse gets sold to Baha Tech, and Fitch Ratings has a new interactive tool in this week’s news roundup.
Nasdaq, AWS offer cloud exchange in a box for regional venues
The companies will leverage the experience gained from their relationship to provide an expanded range of services, including cloud and AI capabilities, to other market operators.
OCC’s security chief on generative AI with guardrails
Clearinghouse looks to scale technology across risk and data operations—but safety is still the watchword.
Bank of America reduces, reuses, and recycles tech for markets division
Voice of the CTO: When it comes to the old build, buy, or borrow debate, Ashok Krishnan and his team are increasingly leaning into repurposing tech that is tried and true.
Waters Wavelength Ep. 313: FIS Global’s Jon Hodges
This week, Jon Hodges, head of trading and asset services for Apac at FIS Global, joins the podcast to talk about how firms in Asia-Pacific approach AI and data.
Project Condor: Inside the data exercise expanding Man Group’s universe
Voice of the CTO: The investment management firm is strategically restructuring its data and trading architecture.
BNP Paribas explores GenAI for securities services business
The bank recently released a new web app for its client portal to modernize its tech stack.
Bank of America and AI, exchanges feud with researchers, a potential EU tax on US tech, and more
The Waters Cooler: Broadridge settles repos in real time, Market Structure Partners strikes back at European exchanges, and a scandal unfolds in Boston in this week’s news roundup.