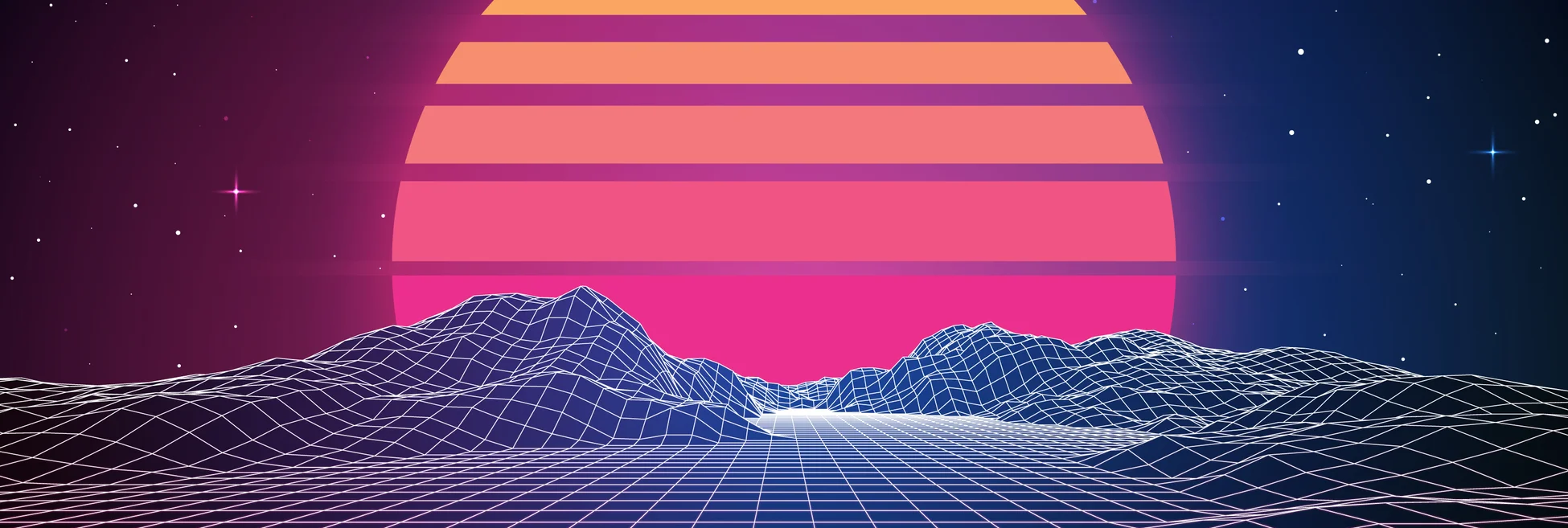
Beyond the 'big red blob': UBS sees future in data mesh for analytics
The Swiss bank has started a journey to implementing mesh data architecture, a new concept for federated data availability in enterprises aimed at getting value out of analytical data.
If you work for a large financial firm, it’s safe to assume that your data architecture is probably what UBS Group CTO Rick Carey calls a “big red blob.”
Like many technologists whose job it is to explain complex concepts to businesspeople, Carey employs some neat formulations, many of which, one senses, have attained the status of in-jokes at UBS.
The “big red blob” quip derives from illustrations in internal slide presentations in UBS’s official colors—the Swiss bank’s logo is black and red. Carey uses the phrase as shorthand for the kind of centralized, monolithic data architectures with which large enterprises have for decades tried to consolidate the data they take in from numerous sources.
These kinds of architectures will be familiar to WatersTechnology readers. Large organizations like banks and asset managers tend to employ various data warehouses and data lakes. Data warehouses are repositories of information standardized and managed for business purposes and fed by extract, transform and load (ETL) pipelines. The warehouse was succeeded by the concept of data lakes, a solution for the era of big data—vast pools of unstructured and semi-structured data, from which users can develop machine learning and AI models, if they can effectively sift through and evaluate this raw data.
Various combinations and hybridizations of these architectures have become buzzwords, especially as organizations are increasingly shifting their entire estates to the cloud. But experts, Carey among them, say these centralized, monolithic constructs can no longer deliver value to enterprises as they scale.
“Traditional data initiatives tend to fail because they have similar characteristics and therefore often look the same—we call it the ‘big red blob.’ The red blob could be a warehouse, a data lake, a lake house, or a lake house on the cloud!” Carey tells WatersTechnology. “What I often tell people when they’re looking at a traditional data initiative diagram is to lean back a little and squint your eyes: You’ll see the same red blob over and over.”
Organizations produce more and more data, and find more and more data from external sources to consume, especially as they grow and evolve. New tools have made it possible to capture more and more information for actionable insight and to improve customer experience.
“The size, sources, and types of data are growing every day. Take the movement of a mouse. It can be tracked to gauge the interest level of a person browsing a webpage: Do they hover? Do they move away? All this information gives us a great understanding of a customer’s experience. The movement of a mouse—think of how much data that is!” Carey says.
Executives are dazzled by the possibilities of all this data, especially as the tools for making use of it—analytics, storage capabilities, processing speed—get better and cheaper, and pour investment into tech and people. These executives like to talk a big game about shifting to a data-driven culture. But throughout financial services and beyond, no one seems to be succeeding with their big data platforms.
Consultancy New Vantage Partners published its 10th annual report on data and AI in January, for which it had surveyed 94 blue-chip corporates—including UBS, as well as JPMorgan Chase, Citi, Wells Fargo, and MetLife. The survey found that while investing in data and AI is growing, businesses are reporting failures on some crucial metrics of success. Of those canvassed, just 19.3% reported that they had established a data culture, 26.5% reported they have created a data-driven organization, and 47.4% reported they were competing on data and analytics.
So why are sophisticated organizations that are cognizant of the value of data and are pumping resources into storage and analytics and engineers failing to realize benefits?
This was a question that, in the 2010s, was troubling consultants at Thoughtworks, an influential software and delivery company known particularly for its central role in the development of the agile methodology.
In studying both successful and failed implementations, one of these consultants, Zhamak Dehghani, observed that enterprise data is siloed, with operational and analytical data in completely disparate “worlds” within the organization. Connecting these two worlds is an inefficient and fragile labyrinth of pipelines, underpinned by data warehouses fed by cumbersome ETL processes and housing data organized under universal, canonical models too inelastic to respond to a dynamic organization, with its proliferation of applications and users, and data.
Dehghani knew that organizational and data architecture is inextricably linked, and she also observed that these data siloes were intermediated by teams of platform engineers who had to become hyper-specialized in the tools that serve big data applications—particularly as clients increasingly want to take these concepts to the cloud. The resulting talent shortage is not news to anyone in this industry, and it is a battle that no business will ever win: As the business grows, it must keep finding more engineers.
Also, according to Dehghani, data engineers are estranged from the producers and the users of the data, and so have little ownership of it; they barely understand how it was generated or the uses to which it will be put. This engineering layer creates a bottleneck for data to flow through the enterprise, and their solutions leave data consumers unsatisfied.
In 2018, Dehghani conceived a new approach, which she detailed later in a blog post. Dehghani wrote that the world needs to shift away from the paradigm of a data lake or a data warehouse to a distributed architecture that considers business domains as owners of data and treats data as a product. Dehghani, anxious to avoid the aquatic metaphors that characterize data management nomenclature, called her new methodology “data mesh.”
Data mesh as an approach is not a technology or a vendor solution or the result of a one-off consultation with a company like Thoughtworks. Rather, it is a tech-agnostic, federated architecture, built using a set of principles, that ensures the on-demand availability of data by users within a business on a peer-to-peer basis.
Thoughtworks did not respond to a request for an interview. But Dehghani has expanded her initial ideas into a book called Data Mesh: Delivering data-driven value at scale, and the first chapter is available for free on the Thoughtworks website.
It’s early days for data mesh, but a small community of evangelists has sprung up, and companies including retailer Zalando and trading platform provider CMC Markets have detailed their experiences of transitioning from the big red blob toward a data mesh architecture.
We mesh well
Data mesh certainly has an enthusiastic convert in Carey, who is excited about the possibilities of the approach.
“You have increasing sources of data and an increasing number of consumers—consumers almost always become producers [of data]. This tends to go beyond the limit of traditional data initiatives. And that’s where mesh data architecture looks to be the best solution because ‘big red blobs’ struggle to keep up with the growth of data and the growth of the consumer,” Carey says.
For Carey, data mesh complements UBS’s existing trajectory to becoming data-driven, which is accelerating under the leadership of CEO Ralph Hamers.
Hamers was brought on to UBS in 2020 as a digitizer. Under Hamers, UBS has started moving more employees to an agile way of working, extending the firm’s construct of small, interdiscplinary teams called “hybrid pods” to more of the business, under a program known as Agile@UBS. Hamers said in the group’s Q1 2022 earnings call that 10,000 staff had so far been shifted to agile under this program.
Late last year, the press reported on a memo in which Hamers told staff that UBS would be creating a new bank-wide team—called AI, Data and Analytics (ADA)—to manage data to use more AI and analytics, with the aim of attracting more wealthy clients in a highly competitive environment.
Carey says data mesh will inform the architecture of ADA.
“Data and consumers are increasing, and methodologies like AI, machine learning, analytics, computational capabilities, GPUs, and the cloud are also increasing. That to us is the sweet spot for a data mesh architecture,” Carey says. “Why do we think this? A data mesh architecture starts with the premise that data can be anywhere—and it already is everywhere.”
Carey uses the metaphor of a screen, such as you might find on a door to keep the bugs out. Or perhaps one could think of it as a fishing net, made up of knots and strings that run between them.
“It’s made of interlocking nodes, which have relationships with each other. In other words, the data is the ‘what,’ the consumer is the ‘who,’ the methodology is the ‘how,’ and the mesh contains the paths that bring all these together,” Carey says.
In a data mesh, the nodes are the products—data as a product is a fundamental principle of this approach. Data is a product not in that it’s something ready for sale, but rather in the sense that the customer’s needs come first in its design and packaging. If data is a product, Dehghani writes, it can be shared directly with users who are peers, such as analysts, avoiding siloing and disintermediating platform owners like engineers.
These data products should be available from a self-serve data platform service—think microservices, but for analytics data rather than applications. On these platforms, a data product is managed along its full lifecycle, while being enmeshed with other data products via relationships defined by code. This allows knowledge graph and lineage across the mesh, and provides the user with a frictionless experience as they look for data products.
The data should be discoverable, addressable, and trustworthy, so it needs to have an owner who is close to it, either as its source or as its main consumer. And so a data mesh is federated and domain-driven, meaning that ownership is the responsibility of the business domain it is associated with, rather than a central authoritative data management layer. The lines between the nodes are relationships formed by the users who access the data products from their respective domains.
Because the domains own their own data, accountability for data is also federated, with domain-specific experts responsible for its quality and integrity.
If the data mesh still seems a little abstract, consider a crude example. An analyst within a bank wants to understand correlations between, say, customer experience and economic events. The analyst wants to look at transaction datasets, social media datasets, and client records. In a centralized paradigm, they would have to make a copy of all these datasets and download those.
Not only does the data quickly balloon to millions of rows and columns; the analyst may also struggle to trust entirely in its quality and integrity, as it has no clear owner and its timeliness may be suspect.
“In the big red blob, data tends to be stored for a long time. Data comes from various sources, which requires the blob to stay up to date. This is a tremendous amount of work. Why not just go to where the data is needed?” Carey says.
In a data mesh, the analyst can discover and access the datasets they need as products all in one place, on the self-serve platform. This is why one of the first things UBS did when building its mesh was to develop a browser, Carey says.
The analyst might make a copy to run a complex training algorithm on it, test out their idea and decide that their hypothesis was wrong, and they need to start again with different data.
“You wouldn’t want to [make a copy] against a production system, but with a mesh you could bring a copy over into a different node for a short period of time. And when done, the mesh can purge the data,” Carey says.
Also, the analyst can trust the quality and integrity of the data, because it is overseen by the people who are most familiar with it. As the analyst pulls this data, they may create their own dataset, which then itself becomes a product and is available for later users. The relationships between the datasets—the ones the analyst has used and the ones they have produced—will be encoded as metadata. Metadata defines the “lines” between nodes, the relationships between one product and another. This metadata contains information about, for example, the size of a dataset or when it was last updated, but also crucially how it combines with other datasets.
This process of building the nodes and encoding the relationships between them isn’t “a free-for-all,” however, Carey says. “It takes work: every line and every node needs to be well-defined as you build the mesh.”
For one, much of the data in an organization is sensitive. Perhaps it is confidential or falls under data protection regulation. Or perhaps a particular group of UBS customers would not care to have their data used in a particular way. Thus, every user in the mesh is permissioned to see only the data they have a business reason to see.
In UBS, the metadata about the nodes is stored in the firm’s DevOps platform, DevCloud. UBS launched DevCloud, which is aimed at speeding up development cycles in the cloud, in partnership with GitLab in 2020. The node is about relationships, Carey says, and that meta description of the relationship between one node and another is what goes into DevCloud. When individuals have permission to access data, they can execute code to grab the data from the mesh and the code goes into DevCloud.
Carey says, however, that UBS does not put the data itself into DevCloud.
“We’ve made a large investment at UBS to create a unified development ecosystem because we treat everything as code, except for data,” he says. “It’s not best practice to put data into a DevOps repository. We don’t put data into DevCloud, but we put everything else there since everything is code.”
Carey says DevCloud is helping UBS implement a data mesh in a much stronger way.
“Without DevCloud, it is more difficult in situations where an analyst is trying to get data from three different places, three different nodes across three different businesses. When you have three different development platforms to get data, it’s not a great experience; for us, it’s all one experience,” he concludes.
Further reading
Only users who have a paid subscription or are part of a corporate subscription are able to print or copy content.
To access these options, along with all other subscription benefits, please contact info@waterstechnology.com or view our subscription options here: http://subscriptions.waterstechnology.com/subscribe
You are currently unable to print this content. Please contact info@waterstechnology.com to find out more.
You are currently unable to copy this content. Please contact info@waterstechnology.com to find out more.
Copyright Infopro Digital Limited. All rights reserved.
As outlined in our terms and conditions, https://www.infopro-digital.com/terms-and-conditions/subscriptions/ (point 2.4), printing is limited to a single copy.
If you would like to purchase additional rights please email info@waterstechnology.com
Copyright Infopro Digital Limited. All rights reserved.
You may share this content using our article tools. As outlined in our terms and conditions, https://www.infopro-digital.com/terms-and-conditions/subscriptions/ (clause 2.4), an Authorised User may only make one copy of the materials for their own personal use. You must also comply with the restrictions in clause 2.5.
If you would like to purchase additional rights please email info@waterstechnology.com
More on Emerging Technologies
Nasdaq, AWS offer cloud exchange in a box for regional venues
The companies will leverage the experience gained from their relationship to provide an expanded range of services, including cloud and AI capabilities, to other market operators.
OCC’s security chief on generative AI with guardrails
Clearinghouse looks to scale technology across risk and data operations—but safety is still the watchword.
Bank of America reduces, reuses, and recycles tech for markets division
Voice of the CTO: When it comes to the old build, buy, or borrow debate, Ashok Krishnan and his team are increasingly leaning into repurposing tech that is tried and true.
Waters Wavelength Ep. 313: FIS Global’s Jon Hodges
This week, Jon Hodges, head of trading and asset services for Apac at FIS Global, joins the podcast to talk about how firms in Asia-Pacific approach AI and data.
Project Condor: Inside the data exercise expanding Man Group’s universe
Voice of the CTO: The investment management firm is strategically restructuring its data and trading architecture.
BNP Paribas explores GenAI for securities services business
The bank recently released a new web app for its client portal to modernize its tech stack.
Bank of America and AI, exchanges feud with researchers, a potential EU tax on US tech, and more
The Waters Cooler: Broadridge settles repos in real time, Market Structure Partners strikes back at European exchanges, and a scandal unfolds in Boston in this week’s news roundup.
Bloomberg rolls out GenAI-powered Document Insights
The data giant’s newest generative AI tool allows analysts to query documents using a natural-language interface.