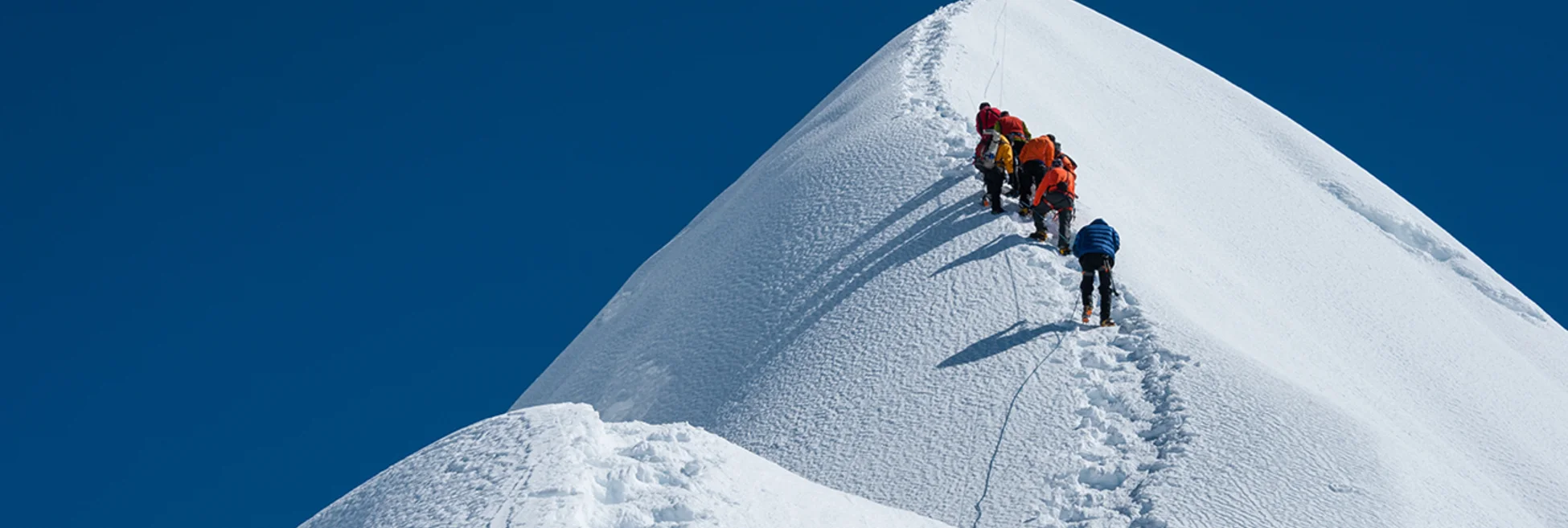
Scaling the Governance Mountain
The perfect data governance strategy is a unicorn, data executives say; yet from setting policies and data quality evaluation to metadata generation, all large financial firms need one. As many firms augment strategy in this area to become more data consumer-centric, Tim Bourgaize Murray examines how fresh approaches to governance require creativity, patience, and technical innovation.
Standing higher than 29,000 feet, Mount Everest is tough—if not impossible—to climb solo, and climbers undertaking the world’s tallest mountain arrive expecting help: Nepalese Sherpas, who play a dual role on Everest’s slopes. On one hand, they plot the climbers’ paths, provide guide-ropes and other protections to make it through the most treacherous passes, guarantee supplies at resting points, and address other technical problems in advance. On the other hand, there is the Sherpas’ mythical quality as protectors of the mountain that is passed generationally, and along with it, an intimate knowledge of Everest’s evolving terrain, of the weather—of a certain feel for the place—that even the world’s best mountaineers cannot furnish for themselves.
Data governance teams at financial institutions might insist this comparison is unfair—and that their job is actually harder than Everest’s famous guides. For decades, data leads—and more recently, chief data officers—have concertedly sought to alter the behavior of data consumers at their firms, and leverage more sophisticated analysis of data management, itself. Like Sherpas, their roles are often several and intertwined: Generate more business value for data users, weed out repeated operational inefficiencies, keep up with compliance, and facilitate broad-based transformation and digitization.
Veterans of the process say getting there is a little like scaling a peak in the clouds, trudging a slow and methodical ascent into what can still be, at times, thin and lonely air. But they are learning there is more than one way to get there.
From Governance to Strategy
Data governance is a tricky concept. If good data management makes information flow within the vast ecosystem of an investment bank or asset manager, then effective data governance—including data quality checks, production of data lineage and policy development—serves the slightly different role of shaping and regulating that flow. These efforts are necessarily defined by client negotiation and buy-in, executive-level support, and the resources to staff a team and run the analytics platforms required to measure how data is used and misused: where, by whom, and to what end? Or, for example, how inaccurate was a calculation, and at what expense?
To do this successfully, those responsible for data governance must be highly attuned to stakeholders within a firm’s business units, and must also be able to influence key back-office operational decisions. Data governance is a key driver in the recent proliferation of CDO roles, has been highlighted by regulators through BCBS 239, which sets out global governance standards for banks, and has surfaced as a component in various post-crisis reporting and risk management requirements. It is also viewed by many firms as the foundation for digital innovation and pushing financial services toward the level of customer-centric data dexterity seen in other advanced industries like pharmaceutical manufacturing.
Yet for all these impetuses and greater structure around the topic, sources say it’s still a struggle. For one thing, traditional models—driving governance with policies and centralized internal regulation of information from the top down, an approach once favored in the early going—don’t match well to the highly dispersed and fragmented organizations at many tier-one firms. For another, the technology to complete data profiling, capture data movement through different processes, and ultimately transform that information into useful tools for users, is still maturing. And third, many within an organization will disagree about what should be prioritized (or will ignore policies altogether).
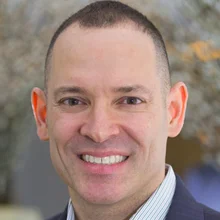
“Data cuts across the enterprise, and regulatory and internal desire for more context around your data, is growing,” says Ken Krupa, chief technology officer at enterprise database provider MarkLogic. “But the traditional ways that we’ve dealt with governance—the models we’ve used in financial services—are flawed when it comes to this area. Following any change along the path—for example, a newly revised regulatory interpretation—from policy down to the data, there are a lot of touch points and friction. Mirroring that evolving policy in the data has thus proven really hard to do.”
Many sources view tackling this as a problem of taking on the impossible, or “boiling the ocean.” As a result, today’s data governance strategies plan their ascent differently: Combine greater agility with new technology that drives institutional consensus.
Micro-Governance
Doing so first means learning from past mistakes, and data veterans suggest that this begins with a tactical perspective on governance, aiming for linkage and enablement, rather than top-down blanket perfection. It’s what Brian Buzzelli, senior vice president and head of data governance at Acadian Asset Management, says is a shift away from the “defense-first CDO posture” to a role that is more of an expert on a firm’s data landscape and operating idiosyncrasies, and less of a technocrat. This is also an implicit and honest acknowledgement of what all data professionals already know: no firm’s data is perfect—far from it—and no amount of governance policy will lead to that perfection. So the goal is already different. “We’re all looking for a point of reference—a place to start without turning the organization upside-down,” he says. “If the perfect model doesn’t exist, you take a specific approach, one that takes into account the culture of the organization and what would be most beneficial for your constituency base.”
For example, Josh Axelrad, market data analysis and management team lead at Credit Suisse, notes that most of his previous work was in the trenches and proved most successful when principles, rather than the vagaries of policy-setting, led the way. “We want data governance layered within our organization, which to us means seeing it from two sides,” he says. “My previous experience was making sure our pricing data has governance around it with data quality checks; now, with related regulations coming in, we understand we need to have a firm-wide picture. So we’re coming at it from both sides: seeing what the interface points are between those functions, and making sure pockets of initiatives across the bank connect up in the right way.”
Likewise, Ellen Gentile, vice president and data quality manager at Sumitomo Mitsui, adds that another feature is devoting “lots of energy” to understanding data consumers’ requirements and animating what is otherwise a dry area. “We took a lot from BCBS 239 in designing what we do, but whether it’s engineering a new reporting process or an entire datacenter, they have to feel engaged in the stewardship process. You always should be able to tell them why they’re there, and how they benefit: Give them that transparency,” she says.
Measuring Up
One explicit way experts are doing just this is by making data governance practices quantifiable, with closer, persuasive and more innovative measurement of data usage and data quality incorporated as a central prong of governance strategy. Indeed, more evidence about the data itself exposes patterns of behavior, allows firms to address processing issues and errors faster, and evaluate the responsiveness within specific datasets to external phenomena. It’s why Gentile insists that data quality testing “must sit within an institution’s data governance functions, not on an island.”
These insights all go to the fundamental question for governance—“how usable is the data”—but they can also be incredibly diverse, testing for reactivity as well as against history. In one illustration, Credit Suisse’s Axelrad says the bank has created a new set of customized rules to evaluate its end-of-day pricing, both for frequency of mark-to-market activities for illiquid instruments, and for observation of term-structure changes. “Again, we’ve done this with an understanding that it’s not ‘one size fits all,’” he says. “We want to create a framework with narrow rules to best understand each asset’s data type.”
A “manufacturing [type] approach” to evaluation is another method to deploy, Buzzelli explains. He says his group at Boston-based Acadian has implemented a new set of quantitative rules applicable to any data point in a SQL-based environment, using a series of novel benchmarks that are applied prior to use. “They include measuring: whether [a data point] falls within a certain standard deviation from the mean relative to data points’ value over specified time; fall relative to certain tolerance given a prior indication; where does it stand [compared] to average for same sampling; Z-scoring for standardizing classes of data, and something we’ve developed called a data-quality benchmark, which is a service-level expectation that uses a consumer-based phraseology about the data,” Buzzelli says. That system is now being extended beyond SQL to other databases.
Lineage Lessons
While these new evaluation tools are critical, they also pose a separate question—and another prong for contemporary data governance: Where should the tools sit within the data management stack to best capture (or explain) what is going on downstream? This tale, sources say, is told with data lineage and by examining the metadata produced in data processes, whether within calculations or report compilation.
“Looking at it end-to-end, you are always examining the health of the data relative to what it is and who uses it—is accuracy the most important dimension, or conformity, or something else?” says Gentile. “But you’re always looking for the elements underneath that make that critical data element accurate, or conformant, and therefore managing those elements is always a data lineage problem.” Agreeing, Credit Suisse’s Axelrad says this also means placing the tools mentioned above—data quality checks and evaluation engines—at different layers, according to the dependencies or branching that goes on upstream. “You’ll only know where to place those once you have your data lineage really laid out,” he says.
Abhijit Shinde, director and head of data governance and quality at New York-based consultancy ITI Data, says that as governance has focused more upon data lineage and wider audiences are attracted to this information, the industry must be sure to provide visualization and lineage reporting options tailored to the technicality of use cases involved. Lineage tools should help further democratize the governance process, that is, and progress is being made here. But MarkLogic’s Krupa argues that this is a spot where institutions’ technology forethought—rather than creativity of assessment and analytics—can have far deeper and lasting impact. And there is more work to be done.
“Whether for metadata, or more recently model risk management, the best way to do data lineage is to have it baked within the data itself, rather than introduced post-process with code and people remapping what’s already been done,” Krupa says. “To have those breadcrumbs already there and lineage available to be queried, and moving from orthogonal overlays to intrinsic part of the data itself, really allows you to capture and even code what you want to do with data governance as a CDO. Today, that’s what our clients tell us: At the point of policy and process below that, and then down to applications and tools, there’s been a lot of churn and improvements. But below all of this it’s at the data layer—where we see the old expectations are still largely intact—that fundamental changes must go next.”
Trust Your Guide
And perhaps therein lies the rub. Indeed, if genuine artificial intelligence (AI) for data governance is the future, the present is decidedly more pragmatic. New data governance strategy gains its success from being more tactical, tailoring to consumers, quantifying data usability and better understanding its geography within the enterprise—all contrasts from the policy-prioritized, centralized approach that came before. “It should be a two-way street,” says Axelrad, describing the new social contract for data. “We expect accountability to policy, but we’re going to give you the toolkit to derive value, too.”
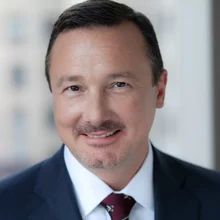
Buzzelli sys no bank or buy-side firm can claim to be doing this in every last corner of its shop. And today, that patience and freedom to experiment seems almost by design—perhaps out of respect for the journey, or the limits of uniformly governing the data at institutions employing tens of thousands of people. Further still, many smaller governance teams are also limited by operational reality—resource constraints and light headcount while “always working through a data management brownfield,” Krupa says.
That’s why Gentile says data governance personnel shouldn’t be surprised to find themselves holding things together with tape, glue, moxie and Excel tables at times. “For a while there, before our platform was stood up, I was the workflow,” she recalls. “But that’s why it’s so important to engage: Governance is about saying to data users, ‘You’re going to be part of the solution. Let me be your Sherpa.”
Only users who have a paid subscription or are part of a corporate subscription are able to print or copy content.
To access these options, along with all other subscription benefits, please contact info@waterstechnology.com or view our subscription options here: http://subscriptions.waterstechnology.com/subscribe
You are currently unable to print this content. Please contact info@waterstechnology.com to find out more.
You are currently unable to copy this content. Please contact info@waterstechnology.com to find out more.
Copyright Infopro Digital Limited. All rights reserved.
As outlined in our terms and conditions, https://www.infopro-digital.com/terms-and-conditions/subscriptions/ (point 2.4), printing is limited to a single copy.
If you would like to purchase additional rights please email info@waterstechnology.com
Copyright Infopro Digital Limited. All rights reserved.
You may share this content using our article tools. As outlined in our terms and conditions, https://www.infopro-digital.com/terms-and-conditions/subscriptions/ (clause 2.4), an Authorised User may only make one copy of the materials for their own personal use. You must also comply with the restrictions in clause 2.5.
If you would like to purchase additional rights please email info@waterstechnology.com
More on Data Management
Growing pains: Why good data and fortitude are crucial for banks’ tech projects
The IMD Wrap: Max examines recent WatersTechnology deep dives into long-term technology projects at several firms and the role data plays in those efforts.
Investing in the invisible, ING plots a tech renaissance
Voice of the CTO: Less than a year in the job, Daniele Tonella delves into ING’s global data platform, gives his thoughts on the future of Agile development, and talks about the importance of “invisible controls” for tech development.
Optiver relies on BMLL market data for quant strategy
The market-maker has built its trading business on top of BMLL’s Level 3 data. But the collaboration is young, and the pair have grand plans to make options the next quant frontier.
Bloomberg expands IBVAL; the SIPs and 24/5 trading; Broadridge’s agentic play, and more
The Waters Cooler: State Street embraces interop, Citi’s CIO outlines the XiNG risk platform, power companies explore alternative nuclear supply options to datacenters, and more.
As costs rise, buy-side CIOs urge caution on AI
Conference attendees encouraged asset managers to tread carefully when looking to deploy AI-driven solutions, citing high cost pressures.
XiNG: Inside Citi’s all-encompassing risk platform
Voice of the CTO: Citi’s chief information officer, Jon Lofthouse, explains how and why the bank has extended its enterprise-wide risk platform so that every trade in any asset class goes through it.
Demand for private markets data turns users into providers
Buy-side firms seeking standardized, user-friendly datasets are turning toward a new section of the alternatives market to get their fix—each other.
LSEG-AWS extend partnership, Deutsche Bank’s AI plans, GenAI (and regular AI) concerns, and more
The Waters Cooler: Nasdaq and MTFs bicker about data fees, Craig Donohue to take the reins at Cboe, and Clearwater closes its Beacon deal, in this week’s news roundup.