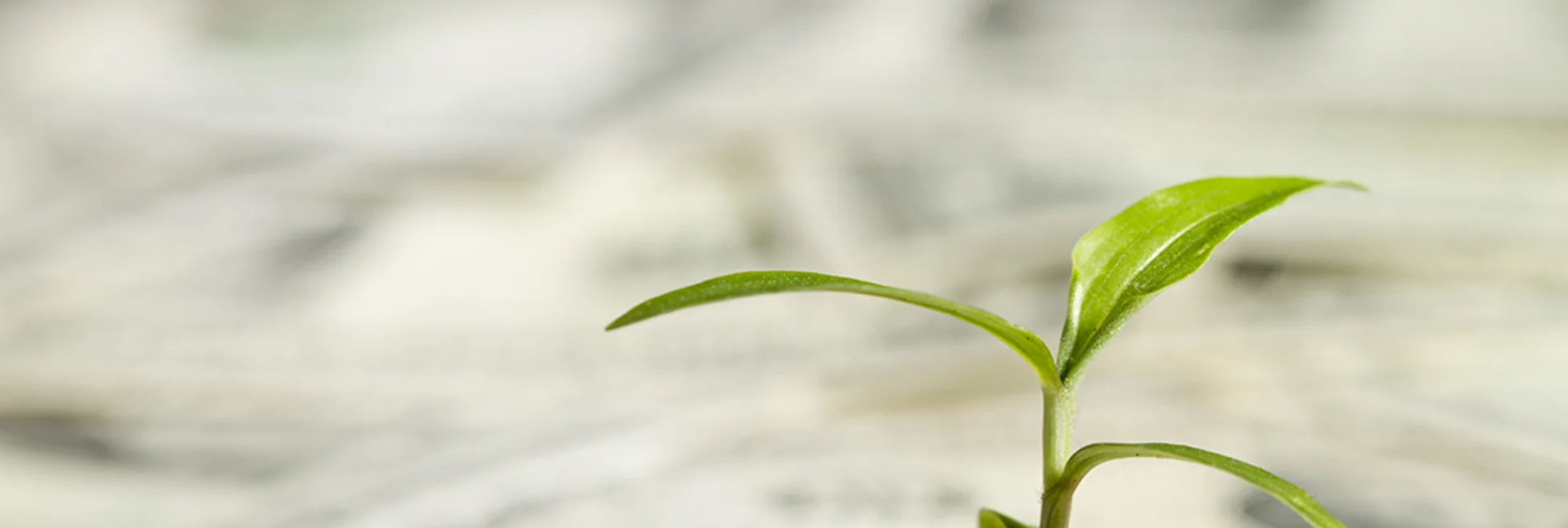
ESG: Turning Green into Green
ESG is booming, but there's room for further development—Waters speaks to executives from banks, hedge funds, asset managers and vendors to explain how this sector is growing and how AI can speed up its development.
Need to know
• Numerous studies have shown that companies with superior performance on material sustainability issues outperform firms with inferior performance on these issues. Additionally, Gen-Xers and millennials, as well as women—each as individual groups and as a combined whole—are more likely to look to invest in ESG-positive companies.
• As passive strategies have gained popularity over active, active managers are having to find new investment vehicles to drive returns. ESG is one potential area for adoption. Assets invested globally in sustainable strategies stood at $22.9 trillion in 2016, up from $18.3 trillion in 2014.
• Artificial intelligence—and specifically machine learning, deep learning and natural-language processing—is helping to distill ESG datasets, which are numerous and are mainly unstructured.
On May 7, a Consolidated Edison (ConEd) substation in Brooklyn dumped thousands of gallons of dielectric fluid into the East River. The failed transformer responsible for the spill contained approximately 37,000 gallons of the mineral oil, which is used to prevent electric discharges. Of that, only about 7,000 gallons have been recovered, with the rest flooding into the water. Allyson Conroy, a Chief Warrant Officer in the US Coast Guard, described it as “a catastrophic failure.”
It will take months—if not years—to fully understand what yet another environmental disaster in the East River will mean for one of New York’s main waterways. It will likely cost ConEd millions in fines and clean-up costs. But after the news hit the wires, something else happened—algorithms found this information and started incorporating it into models and platforms that analyze environmental, social and governance (ESG) data.
While ESG has always been an investment strategy, three things are bringing this sector to greater prominence. First, hundreds of scientific and academic research papers have shown that companies with processes and practices in place that adhere to ESG standards outperform those that do not incorporate ESG standards, or just do enough to keep the regulators off their backs. Second, the amount of information about ESG factors is growing, in the order of 30,000 or more possible factors from millions of sources, both in structured and unstructured form. That, in and of itself, presents a big challenge, which has brought about the third factor: Artificial intelligence (AI) and certain machine-learning techniques have helped portfolio managers and traders distill this data into investable insights.
The market is growing rapidly. According to the Global Sustainable Investment Alliance, in its biannual report, assets invested globally in sustainable strategies stood at $22.9 trillion in 2016, up from $18.3 trillion in 2014. Investment in these strategies grew 33 percent in the US during that two-year period, 12 percent in Europe—the largest market for sustainable investment—and 16 percent in Asia, excluding Japan. Still, challenges and confusion remain, but that can be remedied thanks to more data and platforms targeted specifically at the ESG market.
New Thinking
There are several reasons for this sector’s growing investment. Many will point to the fact that Generation-Xers are replacing baby boomers in the investment space, and with Millennials now entering the workforce, there’s evidence suggesting that these groups care more about sustainability issues and want to invest in companies that back these causes. And others will point out that women are having their voices (and assets) increasingly heard and they—as a group—are more inclined to invest in ESG portfolios.
The evidence supporting these assumptions can’t be discounted. Still, the answer is probably simpler, or at least more to do with money: Firms with superior performance on material sustainability issues outperform firms with inferior performance on these issues. This was the conclusion of a 2015 Harvard Business School study—Corporate Sustainability: First Evidence on Materiality—with researchers finding an estimated 3.39 percent to 8.85 percent annualized alpha improvement for firms with high performance on material sustainability issues as prescribed by the Sustainability Accounting Standards Board (SASB).
Also in 2015, the University of Hamburg, in conjunction with Deutsche Asset and Wealth Management, looked at about 2,200 individual studies—a study of studies, if you will—to examine the entirety of research directed toward the field of ESG and financial performance. The report found that roughly 90 percent of studies find a “nonnegative ESG-CFP (corporate financial performance) relation,” and that, “the large majority of studies report positive findings.” In total, 47.9 percent of vote-counted studies and 62.6 percent of meta-analyses studies found a positive relation between ESG-CFP, while only 6.9 percent and 8.0 percent, respectively, found negative results, with the rest falling between neutral and mixed results.
Mixed Feelings
In the ESG realm, there are also differing opinions as to what truly entails ESG and how seriously firms are taking into account a company’s overall ethos, rather than simply looking at a score. One company that has closely monitored the advancement of ESG is Zevin Asset Management. For two decades, the sole focus of the firm has been on socially responsible investing and advocacy.
Using Zevin’s performance, one can see the relative safety of sustainability during bad times, even if in boom times things aren’t as booming. Zevin uses the MSCI AC World Index as its benchmark for performance. After the internet bubble burst, Zevin’s composite fund lost 21.7 percent from 2000-2003, while the benchmark fell 44.93 percent. During the credit boom (2003-2007), the benchmark gained 153.4 percent while the composite grew by 103.9 percent. And during the credit crisis from the third quarter of 2007 through the second quarter of 2009, the composite declined by only 13.5 percent, while the benchmark declined by 48.9 percent.
Sonia Kowal, president of Zevin, which manages over $500 million in assets, says that while Zevin uses data from third-party providers to help provide insight into companies, it often lacks what her firm is looking for—which goes beyond simply seeking alpha, and deeper into sustainability advocacy.
“Just scoring companies—like the data providers do—doesn’t provide the level of insight that we’re looking for. What we see a lot with more traditional, mainstream investment firms that now purport to do ESG research and integrate it into their strategies, is that they overlay scores and that’s a simplified way of doing things,” she says. “Some ESG issues have a quantifiable impact on company financials, and then other times you need a more qualitative or subjective approach to see if a company is behaving responsibly. So we have a lot of intentionality in the way we use this information—we’re not using it to spot market inefficiencies; we’re interested in the company doing the right thing.”
While Zevin’s ethos will not overlap with the likes of a large bank or asset manager, Kowal has seen growth in the space first hand, and the need for active managers to differentiate their worth compared to passive strategies.
“If you step back and look at the wider asset management industry, what you’re seeing is huge money flows out of active management and into passive products because they’re much, much cheaper. So those active managers that are left have to figure out, ‘What’s my new edge? How can I attract and retain clients? What can we do that passive can’t?’ ESG is a big part of that,” she says. “A lot of them are doing it because they see opportunity there, they see this transfer of wealth to younger clients and they think that’s going to be their thing.”
It’s also important to note that not everyone is sold on the potential of ESG. While retail and larger sell-side institutional shops have been launching products to seize on demand, buy-side firms—and, specifically, hedge funds, as pensions funds have been active in the space in recent years—have been slower to adopt these strategies. Kowal says she hasn’t seen hedge funds enter the space. A CTO of a hedge fund with assets under management totaling over $20 billion, tells Waters that the reason is because the buy-and-hold nature of ESG portfolios doesn’t always lend itself well to hedge fund investors.
“The problem for investment professionals is the horizon,” the CTO says. “Let us agree that those firms will outperform in the long term, but a hedge fund will never get to capitalize on that as it won’t hold the position long enough. You’ll have a tough time convincing your investors not to redeem because in the long term the company will outperform based on ESG factors. I hate to be cynical, but the front office sees it as marketing and pay it lip service, but won’t consider it in their investment process—and probably rightfully so.”
But that’s where AI can also come into play in the future—these tools can sift through massive volumes of unstructured datasets far faster than a human analyst can. They can find and pair potential securities with other similar vehicles that can easily fit into non-ESG-focused investment strategies. They can also be paired with other alternative datasets, which is something the hedge fund community has been actively investing in, says the CTO.
“If you’re concerned about the ‘G’ of ESG—governance—you can relatively easily deploy NLP (natural language processing) to scrape news and management notes and create an algo to rate companies. Investors who use NLP for news and research reports can adapt existing programs for this purpose,” says the CTO. “For ‘E’, there’s satellite imagery analysis that is best applied to environment data—carbon and smoke emission, waste, any ‘excretion’ really. That data can then be normalized, compared to peers and quantified. Getting the data is key. Once you have it, you don’t have to be too accurate to make it useful. And, with time, you will become more accurate.”
Case Study in AI
When talking about ESG and AI, NLP is the main tool right now. One platform that acted immediately after the ConEd spill hit the news wires was TruValue Lab’s Insight360 SASB Edition analytics engine. Most ESG data is supplied by the individual companies and can have a lag time of four months to four quarters, if not more. On the other hand, Insight360, which is built using SASB materiality standards, uses AI to sift through publicly available, but largely unstructured data sources from across the internet—for instance, magazines, trade publications, news sites, thought-leader blogs, a parsed set of social media “experts,” as well as government filings. “We build an army of bots, if you will, that crawl through the internet and find pieces of information that are substantive for the data that we need,” says TruValue’s CEO and cofounder, Hendrik Bartel.
So for ConEd’s spill, Insight360 produced a report, read by Waters, stating that “the Con Edison oil spill lowers ESG score in categories that SASB considers material to financial performance.” While ConEd had previously had a relatively strong ESG profile, the event was damaging, dropping its overall “Insight” score to 71 out of 100, placing it in the 83rd percentile in its industry, electric utilities. This score, which is still pretty good—hence why ConEd had previously been so strong when it came to ESG factors—is a score that considers past results and performance to generate an overall, longer-horizon ESG score.
For many, that will be good enough—it’s a bad result, but overall the company is solid. But for traders looking for more real-time information and, more importantly, a momentum score to incorporate into momentum strategies, its “Momentum” score was a very poor 21 out of 100, placing it in the 32nd industry percentile. From there, users can drill down into more granular detail to examine how the electric company ranks on GHG emissions, human rights and community relations issues, employee health and safety concerns, lifecycle impacts of products and services, and accident and safety management, among others.
Bartel says a big problem in the ESG space is there’s very little consensus between different ratings providers because of a lack of standards beyond what SASB considers and what companies file. And those company filings are slow moving, meaning they generally comprise results from just a few quarters in the past. AI and machine learning—and, specifically, natural language processing and generation, and deep-learning techniques—allow for the ability to create reports and analytics closer to real time than traditional analyst-driven reports.
“ESG is not like credit ratings; there’s no standard behind it. ESG ratings are, by and large, very much human-analyst driven and, as such, they’re very subjective by nature,” he says. “We think that ESG happens every day at a company. This data needs to be updated on a much, much more frequent basis than once a year. That’s where the magic of AI comes in. Our platform can do this reporting within 11 seconds rather than an analyst doing this over the course of a year and reporting after 18 months.”
One portfolio manager at a tier-1 bank says that it started to look at the AI space after several sophisticated buy-side shops, including BlackRock, entered the market. The bank was already using Thomson Reuters’ Eikon database for ESG screening and when it learned that TruValue Insight360 SASB had an Eikon edition, the bank signed up to use the platform as a form of technical analysis to validate its fundamental and valuation analysis, and to quantify risk in the company and overall portfolio.
“I hadn’t seen a way to apply machine learning and deep learning to what I was doing in the portfolio. Some of the really big players, like a BlackRock, started to go into this space, which led me to start looking for opportunities to use AI,” says the portfolio manager. “I have a robust team of analysts to start with. Before TruValue, we basically would conduct a controversy screen and we would do it by doing Google searches or by looking at areas that might be controversial and it was very time-consuming. If you google a company, there’s going to be a lot of information on it and then you multiply that by 30 to 35 companies on an ongoing basis.”
TruValue provides the initial insights, and from there the analysts can dig deeper. To play on a common theme in the AI field, it’s a case of machines augmenting what a human can accomplish by freeing up their time to focus on more critical areas.
In the Data
One of the greatest areas of consternation when it comes to the ESG space is the data. There’s a lot of it, but that’s not totally the issue. Rather, it’s the variety and lack of standardization outside of what SASB considers material. First, as discussed above, ESG disclosures tend to be well in the rear-view mirror. Second, the reports that companies produce for ESG disclosures are often lacking information and there’s limited consistency from company to company. And third, there’s not an industry standard for companies to express how ESG results affect performance.
Finally, market to market, industry to industry, the data and importance therein changes, creating challenges around apples-to-apples comparisons. And since this is a varied and still-evolving space, the strategies for measuring and understanding many ESG metrics are changing all the time—for instance, the way that carbon emissions are monitored and reporting has changed drastically over the last 10 years thanks to new regulations and technologies used to monitor these emissions.
The field of ESG data and index providers is a burgeoning one. There are the stalwarts, such as MSCI, Bloomberg and Thomson Reuters, as well as Sustainalytics, RepRisk and CDP, among many other information providers. But the field is becoming increasingly diverse, with vendors creating all-encompassing ESG platforms, as well as more specialist organizations that focus on either the E, S, or G. [See box “In the Data” for more]
This is another area where AI can prove effective, as a machine is better at making correlations between carbon dioxide emissions, the number of women and minorities on a board, public disclosures, and performance. Again, this is still quite early days. But the growing sea of data is both a blessing and a curse for those looking to enter the space.
Abraham Thomas, co-founder and chief data officer of Quandl, which is an aggregator of alternative data, including ESG, says that there are two aspects of ESG data that make it especially perceptible to machine-learning techniques. First is the sheer volume of information. He says that while stock prices and financial statements can be managed in an Excel spreadsheet—even if that’s not ideal—ESG is so disparate that Excel is rendered useless, noting that some providers have 100 million data points for ESG.
“There’s no way to handle that in Excel—you can’t chart it or graph it or visualize it unless you use big data and machine-learning techniques,” Thomas says.
The next area—once again—comes down to correlations and variety. Looking at the alternative data space as a whole, most of the qualitative datasets produce only a handful of data points when compared to quantitative datasets. ESG is far more varied than most and requires deeper analysis and connections to create investable insights.
“The way these effects play out tends to be complex and nonlinear; it takes time to manage this information,” Thomas says. “It’s not like looking at how the number of people visiting a particular Walmart went up 10 percent, therefore its revenue is expected to go up—it’s much more about complex, fuzzier relationships [between factors], which a machine-learning algo is very good at picking out, whereas a human analyst would just look at it and say it’s noise.”
But the machines aren’t taking over just yet, Thomas adds.
“I would say that the majority of ESG data collectors and data providers aren’t yet using this kind of technique on a regular basis; at this point, most of the folks are just aggregating the data and presenting it as is,” he says. “One thing that is universally true is this: You can’t just take a bunch of raw data and throw AI assets and magically make money—it doesn’t work like that. You need some amount of discretion around what you think is important and how you set the boundaries of your problem. While you might not know the exact weighting of the individual factors in the beginning, you need some human discretion around the boundaries.”
Food for Thought
According to research firm Champions 12.3, for every $1 invested in food waste and loss reduction, companies save $14 in operating cost. Would that make you want to invest in a firm that has an active program to improve its food waste management? Is there a better way to make correlations between those metrics and other ESG factors? It’s early days—and it’s important to not overstate where the industry is at right now—but AI will eventually be able to break down certain walls, which could reap bigger rewards for sustainability causes—simply by showing that ESG can be profitable.
Taaffeite Capital Management is a long-short quantitative hedge fund that is run using artificial intelligence and computational biology algorithms. Its co-founder, Desmond Lun, an MIT PhD and professor of computer science at Rutgers University, tells Waters that the reason why machine learning is being talked about more frequently in finance circles is not because of any great breakthrough, per se, but because of access.
“The thing to understand about machine learning is that there haven’t been any huge conceptual advances over the last several decades—many of the ideas that we’re working with now were already out there a long time ago,” he says. “What’s happened is the maturity of the idea—actually figuring out how to use those ideas in an effective way—and the way in which compute power has gotten exponentially cheaper are the breakthroughs. A lot of these deep neural networks, the basic idea of them has been out there for decades, and now you have the compute power readily available.”
So, NLP can more universally be used for knowledge representation techniques to glean information from scores of documents and then make logical inferences with relatively little compute power necessary, he says. The same can be done with deep learning and pattern recognition. “You can do this with ESG datasets or any other dataset, but you’re trying to recognize the features that distinguish the successful companies from the unsuccessful ones,” Lun says. AI is more efficient at that than a team of humans.
If ESG is simply the result of Millennials rising as baby boomers enter retirement, then this could very much just be a fad. If this increase in investment has more to do with the fact that these are better-performing companies, then active managers will continue to drive asset flow in that direction. If those managers can make better-informed decisions thanks to more data sources, and if machines can help them to distill that information, then they are going to be more likely to stay in the space after making an initial investment.
This also doesn’t mean that firms have to go and build ESG-specific portfolios—rather, this data and the analytics built around this information can be used as an additional set of factors alongside other more traditional datasets to help a portfolio manager make more judicious investment decisions. And if ConEd spills oil into a river, it doesn’t mean that you have to dump that stock—rather, using deeper analytics, you might find that over a longer horizon, the electric company is a good play, even if this accident will hit it in the wallet and hurt its reputation in the short-term. Trading is all about making informed decisions—ignoring ESG is willfully ignorant. And the research is there to prove it.
In the Data, Part II
Five years ago, at its inception, OWLshares was going to be an ESG exchange-traded fund provider. But as its founders began conducting its research, it decided to transition and become an index and ESG score provider instead.
“The process of reviewing data from different providers we found out that there was a big discrepancy if you looked at the scores. [Each vendor] has different ways to create a score: AAA, 1 to 100, 1 to 10, a grade,” says the company’s president and CEO, Benjamin Watson. “If you normalized all of those different scores, you would see that for any given company, their score would vary greatly.”
OWLshares creates a meta score for each company that it provides research on comprising both privately-sourced third-party data and publicly available information in order to create a materiality analysis of the company compared to its peer groups.
“You can’t look at factors in a vacuum,” Watson says. “At any given time there are things happening in a sector, sub-sector or region that could generate relative outperformance or underperformance that are completely unrelated to ESG. We look at ESG factors as one factor among many, and we try to normalize by peer group to try and create equal footing, to [allow users to] isolate the effect of that ESG factor.”
OWLshares is in the process of incorporating AI and machine learning into its platform that will focus on finding the intersection of materiality and ESG data. “The research we are doing is going to be based on peer-group analysis, because from our research, that’s where you get the greatest signals. The only challenge is the scale of the project—bandwidth and manpower, and AI can help with that,” he says.
While OWLshares looks at the full realm of ESG, there are companies like Audit Analytics that have a very focused offering. In this case, Audit Analytics only looks at the “G”—governance disclosures related to regulatory audits and from there they provide market intelligence information to accounting firms and information about the quality of financial reporting to the regulators, public companies, and academics.
“We’ve built out databases that look specifically at corrections that are being disclosed by companies so that users can look back to, say, 2000 and understand every single public company that issued a financial restatement, what the reasons were behind those restatements, and what the impact of those restatements were,” says Joseph Cyr. “If you’re relying on the financials of public companies, you have to be confident that their controls are good for the systems that are producing these financial reports.”
So, for example, earlier this year in its fiscal year 2016 10-K report, eBay disclosed a material weakness in its internal controls over financial reporting, known as an ICFR, specifically around its ability to properly apply tax accounting to its deferred tax asset and income tax benefit accounts. Audit Analytics found that among companies that are required to have an auditor inspect their internal controls, 36 reports—or 17.82 percent of all ineffective attestations—cited tax accounting as one of the reasons for ineffectiveness.
The eBay announcement followed a February 2015 adjustment to correct an $88 million tax error. Following that announcement, the Securities and Exchange Commission issued a comment letter to the retailer requesting additional information about the materiality of the error and its impact on internal controls over financial reporting.
“We’re taking statements that are very qualitative in the financial reports—some of these disclosures about restatements that are very qualitative in nature. But because we’ve developed a taxonomy for tracking these types of corrections and disclosures, someone using our database can very quickly compare to other companies that have had a similar type of disclosure or, in some cases, maybe identify that this is unique to that particular company in that particular industry and do further research on that company…As we see more and more accounting relating issues causing financial meltdowns of companies, I think there’s more and more interest in understanding to what extent do companies disclose these types of statements or have these control issues,” says Cyr.
Only users who have a paid subscription or are part of a corporate subscription are able to print or copy content.
To access these options, along with all other subscription benefits, please contact info@waterstechnology.com or view our subscription options here: https://subscriptions.waterstechnology.com/subscribe
You are currently unable to print this content. Please contact info@waterstechnology.com to find out more.
You are currently unable to copy this content. Please contact info@waterstechnology.com to find out more.
Copyright Infopro Digital Limited. All rights reserved.
As outlined in our terms and conditions, https://www.infopro-digital.com/terms-and-conditions/subscriptions/ (point 2.4), printing is limited to a single copy.
If you would like to purchase additional rights please email info@waterstechnology.com
Copyright Infopro Digital Limited. All rights reserved.
You may share this content using our article tools. As outlined in our terms and conditions, https://www.infopro-digital.com/terms-and-conditions/subscriptions/ (clause 2.4), an Authorised User may only make one copy of the materials for their own personal use. You must also comply with the restrictions in clause 2.5.
If you would like to purchase additional rights please email info@waterstechnology.com
More on Data Management
The Model Context Protocol brings agents to life—along with risk
Waters Wrap: From chat to infrastructure modernization, Anthropic’s MCP offers a ‘bridge’ to agentic AI, but its early days may prove disillusioning.
Agentic AI comes to Bloomberg Terminal via Anthropic protocol
The data giant’s ubiquitous terminal has been slowly opening up for years, but its latest enhancement represents a forward leap in what CTO Shawn Edwards calls, “the way we should talk to the world.”
EU, US consolidated tape efforts pass important milestones
The IMD Wrap: Europe is setting up its first consolidated tapes of data, while the US is revamping its tapes into one. Both initiatives should bring greater transparency and efficiency to the capital markets.
Exchange M&A, US moratorium on AI regs dashed, Citi’s “fat-finger”-killer, and more
The Waters Cooler: Euronext-Athex, SIX-Aquis, Blue Ocean-Eventus, EDM Association, and more in this week’s news roundup.
EDM Council expands reach with Object Management Group merger
The rebranded EDM Council now includes members from industries outside financial services.
As datacenter cooling issues rise, FPGAs could help
IMD Wrap: As temperatures are spiking, so too is demand for capacity related to AI applications. Max says FPGAs could help to ease the burden being forced on datacenters.
Bloomberg introduces geopolitical country-of-risk scores to terminal
Through a new partnership with Seerist, terminal users can now access risk data on seven million companies and 245 countries.
A network of Cusip workarounds keeps the retirement industry humming
Restrictive data licenses—the subject of an ongoing antitrust case against Cusip Global Services—are felt keenly in the retirement space, where an amalgam of identifiers meant to ensure licensing compliance create headaches for investment advisers and investors.