A Blueprint for Alternative Data in Asset Management
UBS Asset Management’s data chief sets out his recommendations for using alternative data in the investment process.
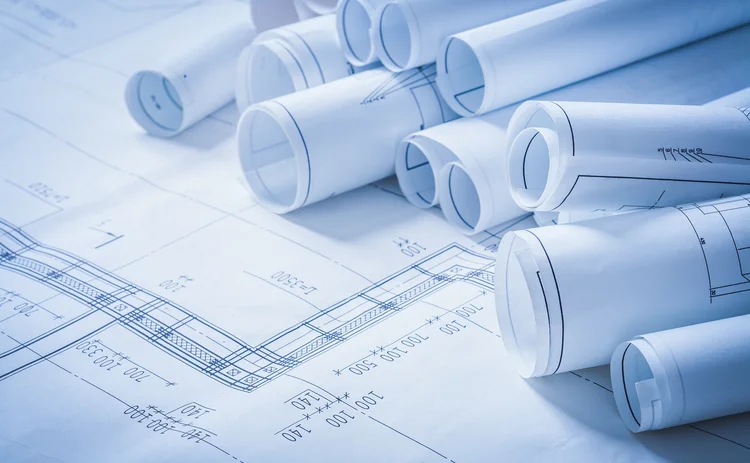
Understanding and implementing alternative data is a difficult task—but it’s an increasingly important one for asset management firms. If properly applied, such data can provide unique insights into economies, sections and companies beyond mere earnings and market information.
Traditional data can be broadly defined as company-specific information, or market data that is aggregated, harmonized and provisioned by data vendors such as Bloomberg, or exchanges such as the NYSE. Alternative data, on the other hand, comes from a wide array of sources, including mobile phone activity, transactional data from credit- or debit-card sales, social media activity, GPS tracking, drone or satellite imagery, and many others.
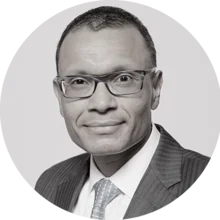
The concept of alternative data initially started with companies trying to improve their own products and services. Examples include Facebook configuring content by its users’ likes and dislikes, and MasterCard analyzing transactions for fraud. These companies soon realized the commercial potential of the data they were collecting, and started selling it to interested buyers. This, in turn, gave rise to third-party providers who collect, process, and supply such data from multiple providers. Examples include credit-card transactions aggregated by Earnest, and satellite image processing provided by Orbital Insight. These developments haven’t been without controversies, and this evolution has raised many data privacy concerns, leading to new regulations restricting the acquisition or distribution of such information without consent.
As more asset management firms continue to explore the use of alternative data, we would like to suggest best practices and recommendations for developing a blueprint for implementation:
Relevance: The application of these capabilities should differ by investment strategy, such as investment style, sector, region, and asset class, among others. The type of data relevant to a long/short equity hedge fund manager will be different from a long-term active asset manager. Signals generated by alternative data can have a short timeframe in which they can be applied, and the signals themselves can have high turnover with changing market dynamics. At UBS Asset Management, we take different approaches for active management, systematic investing and hedge funds. Systematic and quant teams typically like to source alternative data with good history, allowing them to backtest the data through their quant and optimization models, whereas active management teams prefer to get data to support or reject a research hypothesis at a given point of time.
Long-term thinking: Don’t rush it. Research processes and investment philosophies were not developed overnight—they result from decades of academic research and industry experience. A recent benchmarking study, which was sponsored by UBS Asset Management and was conducted by Element22, surveying 20 diversified asset managers representing 20 percent of global assets under management, found that asset managers who are making measurable gains through alternative data efforts started the journey four-to-five years back. The study also indicates a prudent, transparent, informed, and engaged process is required to develop these capabilities for long-term differentiation, with room for iteration and improvement.
Organization and Engagement Model: This is one of the most important aspects, which often gets overlooked. Developing all the scientific and engineering methods possible to utilize alternative data is not going to produce the intended results unless you begin to transform your research process through these insights. It is imperative to create an engagement model that mandates and incentivizes both the producer of such insights, and the research teams who take active interest in evaluating the insights as part of their specific research efforts. Forming dedicated teams within the research function, who focus on insights produced through alternative data, natural-language processing (NLP) and artificial-intelligence capabilities, promotes ideation and that application of insights into traditional research methods.
Talent: You may need skill sets that are not typically found within traditional asset management organizations. Designing IT architectures to support high volumes of data, Agile engineering to process unstructured information, machine-learning skills to develop mathematical and statistical models to extract unique trends and insights are just some examples of requisite skills for any successful application. Given the continuous evolution in these capabilities, you will want to invest in a team that has a similar long-term focus, with the agility to produce near-term outcomes. A growing community of mathematicians, statisticians, and data scientists are joining all sectors, from financial services to healthcare, telecommunications, retail and media, not just technology firms.
Data: Before you go on an alternative data buying spree, give serious consideration to all of the internal data you have at your disposal, including thousands of research documents, transactional activity, historical market data and other information. We are discovering useful applications of internal data, from generating unique NLP signals from research documents, to predicting operational errors by analyzing operational-process metrics. On the external alternative data side, we have experienced success with structured data, such as combining credit-card sales data with fundamental data, including earnings and revenue filings. On the unstructured data side, we are finding numerous applications ranging from sentiment indicators in earnings transcripts and news, to the summarization of research content from thousands of research reports.
Platform: Leveraging such a wide variety of data and modeling capabilities will require a scalable and flexible platform. This platform will need to respond to multi-factor backtesting, and be able to handle the requirements of NLP. Firms should start early in planning for such a platform. Beyond the technical capabilities, it will require data governance, model governance, model monitoring, personally identifiable information (PII) data considerations and the like. UBS Asset Management is deploying a hybrid cloud strategy, with the alternative data research platform to be fully cloud-enabled.
To summarize, there are a wide array of alternative datasets becoming available, but improving research and alpha through these datasets requires a thoughtful and long-term approach. We hope our colleagues across the industry will find these considerations helpful in successfully harnessing emerging data, and data-science capabilities within their firms.
Suvrat Bansal is the chief data officer and head of innovation for UBS Asset Management.
Only users who have a paid subscription or are part of a corporate subscription are able to print or copy content.
To access these options, along with all other subscription benefits, please contact info@waterstechnology.com or view our subscription options here: http://subscriptions.waterstechnology.com/subscribe
You are currently unable to print this content. Please contact info@waterstechnology.com to find out more.
You are currently unable to copy this content. Please contact info@waterstechnology.com to find out more.
Copyright Infopro Digital Limited. All rights reserved.
As outlined in our terms and conditions, https://www.infopro-digital.com/terms-and-conditions/subscriptions/ (point 2.4), printing is limited to a single copy.
If you would like to purchase additional rights please email info@waterstechnology.com
Copyright Infopro Digital Limited. All rights reserved.
You may share this content using our article tools. As outlined in our terms and conditions, https://www.infopro-digital.com/terms-and-conditions/subscriptions/ (clause 2.4), an Authorised User may only make one copy of the materials for their own personal use. You must also comply with the restrictions in clause 2.5.
If you would like to purchase additional rights please email info@waterstechnology.com
More on Data Management
Insurers deny cyber premiums are rising
Contrary to banks’ complaints, underwriters and brokers claim current market for policies is soft.
Cutting through the hype surrounding the FDTA rulemaking process
A bill requiring US regulators and institutions to adopt a machine-readable data framework for reporting purposes applies to entity identifiers, but not security identifiers, in a crucial difference, writes Scott Preiss, SVP and global head of Cusip Global Services.
The IMD Wrap: Quality drivers—the sticks and carrots accelerating the data quality race
Like a Formula One Grand Prix, data management is a race that can be won or lost. And just as each race is part of a larger F1 championship that pays large sums of TV money to the winning team, winning or losing one race can contribute to winning or losing an endgame with much more at stake.
Northern Trust offers internal fund accounting, data tools to clients
Regulations and a mandate to enhance quality and transparency in a bid to improve the investor experience are pushing buy-side firms to have more oversight of their third-party providers.
$135.6m fines prompt Citi to modernize infrastructure, controls
The bank was hit with a combined $135.6 million fine on Wednesday for failing to resolve “longstanding internal controls and risk issues,” amid continued internal work across the enterprise.
SocGen pushes data, analytics use cases for SG Markets
The bank is letting a handful of clients experiment with its proprietary data and models to inform their research.
Finra clears hurdle with CAT launch, but several others remain
Two major components of the consolidated audit trail are now in place. But wrangling over the CAT’s future continues.
Ace high or busted flush? Digital Asset’s mixed fortunes mirror DLT adversity
The vendor hoped to remodel post-trade using blockchain technology—and it still might—but its bumpy progress raises questions over the future of DLT in finance.