After Building an AI Black Swan Detector, Compellon Mulls Path Forward
The California-based company has built a quantitative analysis engine using machine learning to help score sell-side analysts and search for potential black-swan events.
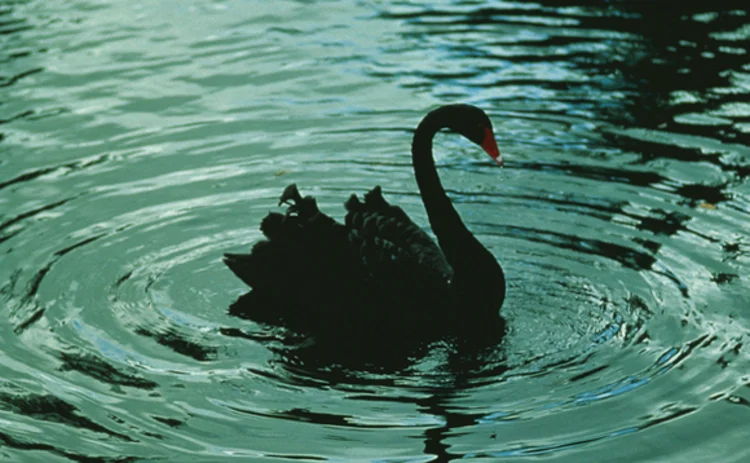
It’s an area that Hamidi had been exploring for years, his background being in building analytics and quantitative research platforms at various asset managers and hedge funds for close to two decades. He was immediately impressed with the platform, which was built by Compellon co-founder and chief data scientist, Dr. Nikolai Lyashenko. Rather than settle down on the East Coast, he decided to join the Laguna Hills, Calif.-based technology provider to build, from the ground up, its capital markets practice.
Hamidi says that what drew him to the company was a certain level of freedom to practice his craft. For years he had been limited by specific tools—R and Matlab for programming, and linear regressions and neural nets that had been around for a long time, but didn’t achieve what he wanted to do in quantitative analysis. By contrast, the Compellon platform is built using the Scala language, with the backend running on Amazon Web Services, enabling it to store and drill into huge datasets, and to scale as needed.
It uses supervised machine learning, the end result being that it helps alert a portfolio manager (PM) like Hamidi to anomalies or to previously unconnected data points, says Marc Bir, Compellon’s chief technology officer. “We can’t tell you what will happen if it’s never happened before,” he says. “The engine itself is data agnostic; we don’t care where the data comes from. It’s looking for drivers of patterns that are causing a certain behavior.”
Compellon’s engine allows the firm to both create predictive measurements for when a high-sigma or black swan event might hit over a 5-, 10- or 20-day window, as well as allow them to score sell-side analysts.
Its S3 signal, which stands for Sell-Side Score, looks at every analyst-generated report for every stock that Compellon has access to, and looks at several aspects of the report—such as price, upgrades and downgrades, and earnings-per-share—to make a judgement on which analysts and data providers are the best and which analysts and data providers may be gaming their numbers. The engine’s rigor, in some cases, has already borne fruit.
“In the case of one [data provider], the AI picked up discrepancies in real time,” Hamidi says. “When we fed their data into the AI and began updating them daily as the company published them, over a period of four months we noticed they would change a model and re-publish historical performance based on the new formula. We ripped apart their entire data catalog and, within a few weeks, had reconstructed their models and then commenced on improving them by introducing alternative and various time series data. Ultimately [we came] to the conclusion that data vendors trying to sell into the AI space have to be quality checked and re-checked before one can trust the data has not been manipulated, or otherwise corrupted.”
Bir says that you can throw 20,000-plus variables at the engine and it will say that, out of this sea of data, here are two dozen variables that show some form of stability, here’s how they have shifted over time and this is how they’re all related. In this way, the platform uses machine learning to help a PM not only to identify areas to drill into in greater depth but to see how these data points can interact with other projects on the trading desk.
“We let the data tell us the model structure, rather than the PM choosing the model structure. As part of that, since we have the data tell us, we get a better understanding of the data, as well as understand how our models are created,” Bir says. “That allows us to know how to adapt it as data changes and as we discover mistakes within our own predictions.”
To Sell or Not to Sell?
Perhaps ironically, the biggest question facing Compellon now isn’t one which can be answered by its engine. Rather, it’s a question of where it goes from here in terms of using it.
The company was founded in 2011, with a focus on big data and predictive analytics for sales and marketing teams in telecoms and insurance, among other areas, delivered using a software-as-a-service (SaaS) model. But it wasn’t until Hamidi joined that it decided to enter the capital markets space.
While the plan might have originally been to use a similar SaaS model for its markets practice, Compellon decided instead on a more protective path—the firm instead uses a subscription model to gain insights from the platform, which are gathered and analyzed within its own walls.
“In the capital markets space specifically, so far we have not allowed any company to take a license to the product, because we’re doing all the research internally,” Hamidi explains. “We have to keep it ring-fenced until we decide whether we want to go after the subscription model in this space or we’re going to wrap this into some sort of pure alpha-generating platform that we use internally with some sort of a hedge fund.
“So the question becomes: Do we use it ourselves or do we actually go talk these funds and let them use it, or do we create some sort of joint venture? We haven’t figured that out yet,” he says.
Further reading
Only users who have a paid subscription or are part of a corporate subscription are able to print or copy content.
To access these options, along with all other subscription benefits, please contact info@waterstechnology.com or view our subscription options here: http://subscriptions.waterstechnology.com/subscribe
You are currently unable to print this content. Please contact info@waterstechnology.com to find out more.
You are currently unable to copy this content. Please contact info@waterstechnology.com to find out more.
Copyright Infopro Digital Limited. All rights reserved.
As outlined in our terms and conditions, https://www.infopro-digital.com/terms-and-conditions/subscriptions/ (point 2.4), printing is limited to a single copy.
If you would like to purchase additional rights please email info@waterstechnology.com
Copyright Infopro Digital Limited. All rights reserved.
You may share this content using our article tools. As outlined in our terms and conditions, https://www.infopro-digital.com/terms-and-conditions/subscriptions/ (clause 2.4), an Authorised User may only make one copy of the materials for their own personal use. You must also comply with the restrictions in clause 2.5.
If you would like to purchase additional rights please email info@waterstechnology.com
More on Emerging Technologies
EU banks want the cloud closer to home amid tariff wars
Fears over US executive orders have prompted new approaches to critical third-party risk management.
Growing pains: Why good data and fortitude are crucial for banks’ tech projects
The IMD Wrap: Max examines recent WatersTechnology deep dives into long-term technology projects at several firms and the role data plays in those efforts.
Waters Wavelength Ep. 317: Bitdefender and Transilvania Quantum
This week, Bitdefender’s Adrian Coleșa and Transilvania Quantum’s Sorin Boloș join to discuss security vulnerabilities in quantum computing.
Investing in the invisible, ING plots a tech renaissance
Voice of the CTO: Less than a year in the job, Daniele Tonella delves into ING’s global data platform, gives his thoughts on the future of Agile development, and talks about the importance of “invisible controls” for tech development.
Evalueserve tames GenAI to boost client’s cyber underwriting
Firm’s insurance client adopts machine learning to interrogate risk posed by hackers
Waters Wavelength Ep. 316: Finbourne Technology’s Toby Glaysher
This week, Toby Glaysher, chairman at Finbourne Technology, joins the podcast to discuss the asset servicing industry.
State Street’s interop play for FX and easing technical debt
Waters Wrap: About six years ago, State Street partnered with Interop.io to tie together its GlobalLINK suite of platforms. Anthony explores how this plays into the “reuse” mantra.
As costs rise, buy-side CIOs urge caution on AI
Conference attendees encouraged asset managers to tread carefully when looking to deploy AI-driven solutions, citing high cost pressures.