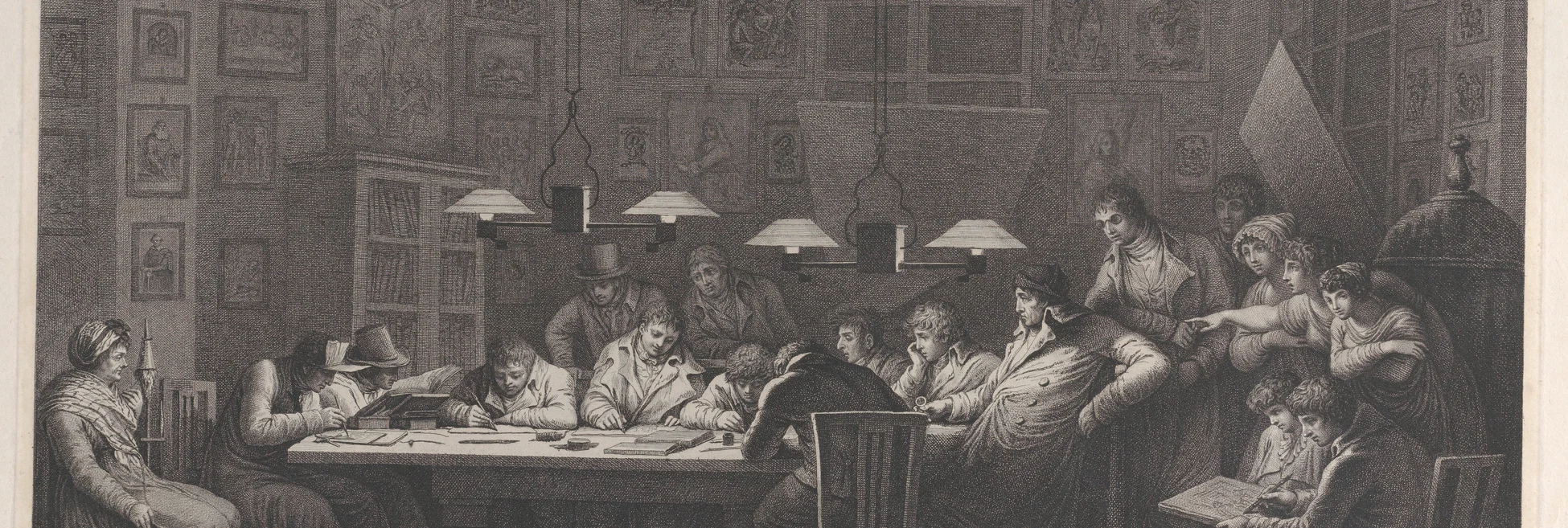
Waters Wrap: Causal Machine Learning Represents Next Evolution of AI (Plus ESG & Innovation Issues)
Anthony looks at an interesting project using causal inference by IBM and Refinitiv, and what this latest evolution of machine learning could mean for innovation in the capital markets in the future.
The inaugural WatersTechnology Innovation Exchange is behind us. Lots more ESG, machine learning, and innovation strategy material from the last week to discuss, but be sure to check out some of our other coverage of low-code applications, zero-trust architectures, and the Open Digital Rights Language (ODRL)—these just might be the hot topics at Innovation Exchanges of the future.
Cause & Effect
The Covid era is a proving ground. For example, alternative data providers have been espousing the wonders of their unique datasets, but if you can’t prove that your datasets are valuable in these volatile markets, the oxygen in the room is going to escape real quick.
Now stretching into its ninth month here in the US, the pandemic has also turned up the heat on machine-learning models that have historically relied on correlations between different types of datasets. Some very interesting work underway by IBM and Refinitiv could help brace these models for the future.
To address correlational problems that challenged firms even before the pandemic, Refinitiv Labs and MIT–IBM Watson AI Lab have been working together on an emerging field of machine learning study called causal inference.
“Rather than trying to predict something based on correlations, you are really trying to dig in and understand those cause-and-effect relationships both through sophisticated statistical tools, but also in many cases through interventions,” David Cox, IBM director of the MIT–IBM Watson AI Lab, told Hamad Ali.
It’s a cool-sounding project, though still in its very early days, and it won’t yield new offerings for Refinitiv customers just yet. But this is a field that I think capital markets firms will have to explore. To better understand causal machine learning, though, it’s important to understand the evolution of the broader ML field and the trends driving advancements there.
The concept of deep learning, which relies on complex neural networks, has been around for decades, but in 1982 it had its own renaissance. The problem it then ran into was that the hardware wasn’t there to commercialize this highly-advanced subset of machine learning. It wasn’t until 2012 that neural networks started to evolve as GPUs emerged and CPUs grew exponentially faster.
There was finally enough power to make these neural nets run efficiently, and that opened Pandora’s box. Data scientists began looking at other problems that were previously inaccessible around reinforcement learning and more advanced and complicated statistical methods. It was only a matter of time until capital markets firms started experimenting with deep learning.
Causal machine learning is the next link in a long evolutionary chain that started with statistics, which matured into data science, which then became machine learning, then deep learning, then reinforcement learning, and then statistical learning. It’s an advancement built upon the needs of the more modern methods in modeling technologies. At its core, you can crunch more numbers more efficiently. Sounds pretty useful for finance, no?
Now, please understand one thing: I have a B.S. in journalism from Plattsburgh State University—emphasis on B.S. So I’m just trying to do my best to parrot the words of people much smarter than I. With that stated, as I understand it, causal machine learning comes down to meta learning.
Models have advanced enough where quants are looking for higher-tier problems to solve. One area of optimization is to actually influence the machine-learning algorithms as they run to make them more efficient. After all, causal inference is not a machine learning model, itself; it’s a series of different types of models that learn about the machine-learning process and influence that process along the way to help it make smarter decisions.
Anytime someone uses the word “meta” to describe something, I roll my eyes. It’s an overloaded term that has become cliché, but it does have a place in finance. Metadata is literally data about data. So maybe you have a core dataset, the metadata is, say, the number of rows contained within the dataset. It’s not part of your core dataset, but you can now derive data about your core dataset. It’s learning about how you learn.
Meta learning helps data scientists learn about how the model learns; it tries to ascertain what it can do to make your learning process more efficient.
Causal inference (and things like stochastic programming techniques) roughly fall into meta learning. If companies like IBM and Refinitiv are successful with their experiments, it would allow quants to use more resilient machine-learning models.
“We are living through an era now where all of the correlations [in machine learning models] sort of broke overnight,” IBM’s Cox told Hamad.
A breakthrough in the field of causal inference could further democratize machine learning, as banks and asset managers are struggling with explainability issues and models that struggle under adverse conditions. Financial services firms don’t need to invent their own power drill—they just need a power drill with a couple of different settings and swappable bits for different scenarios. Causal inference could do that for machine learning.
The Evolution of Trading Tech Innovation
Even before Covid-19 took hold, senior executives at banks, asset managers, exchanges, and vendors, alike, where having serious conversations about what “innovation” would look like at their organizations: Would they be aggressive in hiring the best-and-brightest engineers and data scientists? How would they consider bleeding-edge technologies like quantum computing? Would they focus their efforts on regulatory needs, trading platforms, or user front ends? Would they look to develop these new technologies in-house, or buy a vendor’s platform or tool?
The coronavirus pandemic has only complicated those questions, as banks—at least—are diverting IT budgets toward remote-working tools, away from more innovative, longer-term projects. But as I wrote previously, I don’t necessarily think that this shift is solely due to Covid; rather, I think that banks and asset managers are increasingly using something of a hybrid model when it comes to developing unique solutions.
Moonshot projects are still in the works at trading houses across the globe, even the ones that were unprepared for Covid-19. It’s just that those firms are, and have been, relying on vendors to do the heavy lifting. As I wrote previously, rather than build a Charles River-esque OEMS, State Street goes and buys the company. RBC doesn’t build an operating system to pop its internal OMS into, it partners with OpenFin. Schroders doesn’t develop sophisticated natural language processing models for sentiment analysis, they leave that to a specialist like Owlin. Banks don’t build video conferencing technologies, they license tech from Symphony.
This is also leading financial firms to embrace Innovation Labs and similar incubation programs. As Max Bowie reported this week, BNY Mellon has launched a fintech Accelerator Program to identify and incubate early-stage technology companies building solutions to issues deemed critical challenges by the custodian and its clients.
The idea for the Accelerator Program came after the bank’s recent investment in Kingfield Corp., a provider of an AI platform for improving client service for custodians. BONY brought the company into its offices, enabling its own product teams to help the vendor refine its platform. The Accelerator Program will now look to “industrialize” that process and apply it to more companies.
Why invest in an army of data scientists—why invent your own power drill, if you will—when you can let specialists do the expensive grunt work and then make those specialists part of your own personal toolbox?
What BNY Mellon is doing also reminds me of another article Max wrote: What are banks going to do with their office-space footprints in a post-Covid world? His story pondered whether all this unused office space could become like “WeWork” sites for fintech startups. Investing in, and helping grow smart startups is the way forward for banks (and I think eventually asset managers will follow this lead). If done correctly, that expensive office space could be a useful lure for newcomers.
Green Wave
Again, the pandemic is a proving ground—not just for new forms of machine learning or alt data, but for even an old(er) friend, ESG.
For several years now, sustainability inflows have been increasing. It would appear that Covid-19 is not only not hindering the ESG market, it is helping to show the value of sustainable investing. As Antonia Lim, head of quantamental investments at Schroders, writes, “in the first half of 2020, £70 billion ($89.5 billion) of net global inflows poured into investments flagged ESG. Meanwhile, other mutual and exchange-traded funds—excluding money market funds—lost almost five times that amount. … Rather than retrenching from ESG during the uncertainty brought by the Covid-19 pandemic, investors stuck with it.”
As investment managers increasingly embrace ESG, there’s going to be added pressure to bring standards and greater transparency to this expansive and varied field.
Recently, Joanna Wright spoke with CFTC commissioner Rostin Behnam about a new report addressing climate risk and sponsored by the regulator. It’s the first in-depth look by a financial regulator at the systemic risk that climate change could pose to the US financial system.
The 196-page report makes a litany of recommendations. It says that US financial regulators should collect better data and strengthen their analytics for quantifying climate risk (and to make sure the private sector does the same). It also says that regulators should support the development of standardized systems and taxonomies for risk.
It’s important to note, though, that while the CFTC subcommittee involved in the report voted unanimously to adopt the recommendations, the vote only sets the tone for future policy discussions, but does not have an immediate effect on financial institutions.
So it’s a first step. Whether or not it serves as a backbone to future rules that fall in line with the EU’s stricter climate-related regulation—or whether it’s a precursor to toothless mandates—will likely come down to who wins the US presidential election in November.
The story’s image is Giulo Tomba’s “Rosaspina’s drawing academy”. Courtesy of The Metropolitan’s Museaum of Art’s Open Access program.
Further reading
Only users who have a paid subscription or are part of a corporate subscription are able to print or copy content.
To access these options, along with all other subscription benefits, please contact info@waterstechnology.com or view our subscription options here: https://subscriptions.waterstechnology.com/subscribe
You are currently unable to print this content. Please contact info@waterstechnology.com to find out more.
You are currently unable to copy this content. Please contact info@waterstechnology.com to find out more.
Copyright Infopro Digital Limited. All rights reserved.
As outlined in our terms and conditions, https://www.infopro-digital.com/terms-and-conditions/subscriptions/ (point 2.4), printing is limited to a single copy.
If you would like to purchase additional rights please email info@waterstechnology.com
Copyright Infopro Digital Limited. All rights reserved.
You may share this content using our article tools. As outlined in our terms and conditions, https://www.infopro-digital.com/terms-and-conditions/subscriptions/ (clause 2.4), an Authorised User may only make one copy of the materials for their own personal use. You must also comply with the restrictions in clause 2.5.
If you would like to purchase additional rights please email info@waterstechnology.com
More on Data Management
EU, US consolidated tape efforts pass important milestones
The IMD Wrap: Europe is setting up its first consolidated tapes of data, while the US is revamping its tapes into one. Both initiatives should bring greater transparency and efficiency to the capital markets.
Exchange M&A, US moratorium on AI regs dashed, Citi’s “fat-finger”-killer, and more
The Waters Cooler: Euronext-Athex, SIX-Aquis, Blue Ocean-Eventus, EDM Association, and more in this week’s news roundup.
EDM Council expands reach with Object Management Group merger
The rebranded EDM Council now includes members from industries outside financial services.
As datacenter cooling issues rise, FPGAs could help
IMD Wrap: As temperatures are spiking, so too is demand for capacity related to AI applications. Max says FPGAs could help to ease the burden being forced on datacenters.
Bloomberg introduces geopolitical country-of-risk scores to terminal
Through a new partnership with Seerist, terminal users can now access risk data on seven million companies and 245 countries.
A network of Cusip workarounds keeps the retirement industry humming
Restrictive data licenses—the subject of an ongoing antitrust case against Cusip Global Services—are felt keenly in the retirement space, where an amalgam of identifiers meant to ensure licensing compliance create headaches for investment advisers and investors.
LLMs are making alternative datasets ‘fuzzy’
Waters Wrap: While large language models and generative/agentic AI offer an endless amount of opportunity, they are also exposing unforeseen risks and challenges.
Cloud offers promise for execs struggling with legacy tech
Tech execs from the buy side and vendor world are still grappling with how to handle legacy technology and where the cloud should step in.